Supported Software
Life Science & Molecular Dynamics
RoseTTAFold
Accurate prediction of protein structures and interactions using AI and a three-track neural network.
Capabilities & Features
Protein Structure Prediction
Use deep learning to quickly and accurately predict protein structures based on limited information.
Three-Track Neural Network
Simultaneously consider patterns in protein sequences, how a protein’s amino acids interact with one another, and a protein’s possible 3D structure.
Model Building
Build deep learning models of complex biological assemblies in a fraction of the time previously required.
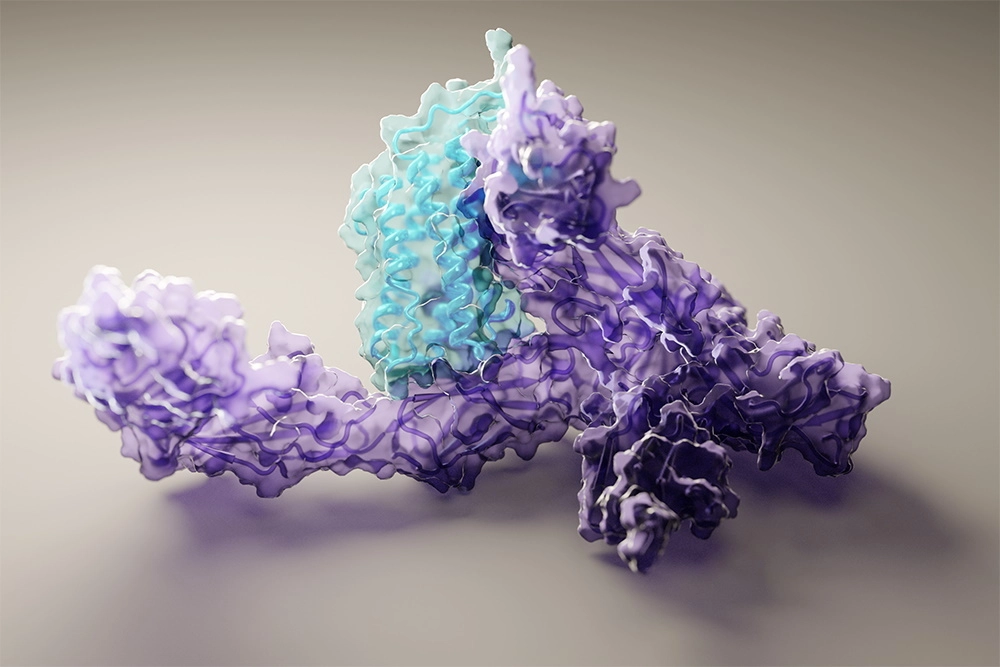
Why Use RoseTTAFold for Protein Structure Predictions?
Without the aid of RoseTTAFold, it can take years of laboratory work to determine the structure of just one protein. With RoseTTAFold, a protein structure can be computed in as little as 10 minutes on a single GPU system. It can solve challenging x-ray crystallography and cryo–electron microscopy modeling problems, provide insight into protein function in the absence of experimentally determined structures, and rapidly generate accurate models of protein-protein complexes.
RosettaCommons
RosettaCommons makes close collaboration between laboratories the norm, even with single code modules. This allows for rapid sharing of enhancements and promotes the values of team science. It has a unique agreement among member universities in which the source codes belong to the RosettaCommons members and is a collaborative effort among research institutions—a model that promotes shared development and discoveries.
CASP
Deep learning approaches to the prediction problem that rely on genomic data have become increasingly popular in the last few years. To catalyze research and measure progress on the newest methods for improving the accuracy of predictions, a biennial global competition called CASP (Critical Assessment of protein Structure Prediction) was established in 1994 and has become the gold standard for assessing predictive techniques.
CAMEO
CAMEO (Continuous Automated Model EvaluatiON) continuously applies quality assessment criteria established by the protein structure community. Since the accuracy requirements for different scientific applications vary, there is no one-size-fits-all score. CAMEO, therefore, offers a variety of scores—assessing different aspects of a prediction (coverage, local accuracy, completeness, etc.) to reflect these requirements.