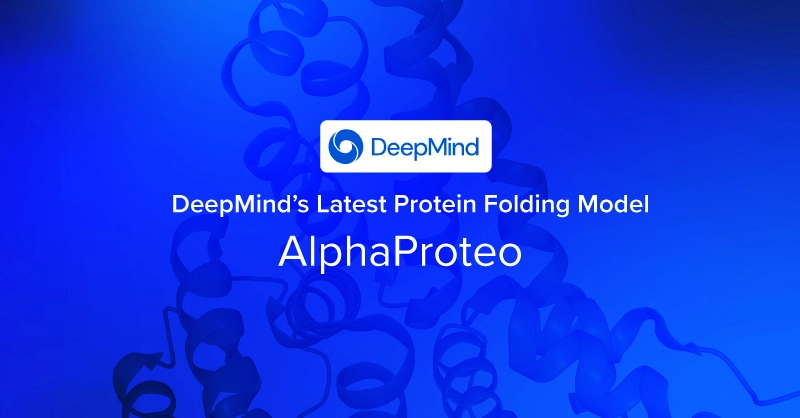
Introduction
In 2020, DeepMind released their revolutionary technology AlphaFold, an AI-powered model for predicting the 3D structure of proteins based on their amino acid sequences. This breakthrough achieved unprecedented accuracy at 80% confidence, revolutionizing the life science industry and propelling research to new heights.
Since then, many research disciplines have incorporated AI protein folding algorithms into their workflows for generating novel designs, validating structural predictions, and testing interactions. Following the successful releases of AlphaFold2 and AlphaFold3, along with a public database containing over 200 million predicted protein structures, DeepMind and Google have introduced AlphaProteo—a model specifically engineered to predict protein binding.
AlphaProteo - Predicting Protein-to-Protein Binding
The AlphaFold group described AlphaProteo as a new system for generating protein-binding protein designs. Unlike previous offerings, AlphaProteo was developed specifically to model and design protein-protein interactions with an emphasis on binding from the start.
Unlike making use of an AlphaFold model plus clever tricks to predict binding (like a long flexible glycine linker connecting two protein sequences of interest), AlphaProteo builds models to deal with protein complexes with ligands, multiple chains, or short peptides.
Notably, it was designed specifically to enable protein engineers to specify a target protein and a specific epitope or residues present on a particular part of a folded protein's surface with regards to movement. These movements are crucial for understanding how proteins perform their functions in the body, such as catalyzing reactions, signaling between cells, or binding to specific molecules. Developing a protein binder designed to tightly target another protein is an extremely difficult problem but particularly useful for a wide range of applications.
AlphaProteo was trained on experimentally determined protein structures from the Protein Data Bank (PDB), as well as over 100 million predicted structures generated by AlphaFold. For evaluation, they focused on only eight targets. Most of these are of particular interest for their prominent role in human health and disease. The percentage represents the number of successful binders generated to bind to the target validated in a wet lab with cryo-EM and x-ray crystallography.
Name | PDB ID | Importance | Success? |
BHRF1 | 2WH6 | Oncogenic protein from Epstein-Barr virus; can slow or stop tumor growth. | ✓
88% success with v1 model |
SARS-CoV-2 spike protein (SC2RBD) | 6M0J | Involved in cell surface binding and invasion. Disrupting spike protein binding can block COVID infection. | ✓
29% success with v1 model and improved filters |
Interleukin-7 Receptor (IL-7RA) | 3DI3 | Cell surface receptor on lymphocytes, treatment target for some leukemias and HIV. | ✓
24.5% success with v1 model |
Interleukin-17A (IL-17A) | 4HSA | Secreted protein that triggers inflammation and target in treating autoimmune disease. | ✓
14.3% success with v1 model |
Tropomyosin Receptor Kinase A (TrkA) | 1WWW | Nerve growth factor involved in autoimmune disease and target in treating chronic pain | ✓
8.1% success with v2 model |
Programmed Death Ligand (PD-L1) | 5O45 | Cell surface receptor involved in regulating immune cell proliferation, target for treating some cancers. | ✓26.5% success rate with v2 model |
Vascular Endothelial Growth Factor A (VEGF-A) | 1BJ1 | Secreted growth factor that controls angiogenesis (blood vessel formation), e.g., used by cancer cells to recruit blood supply and a target in treating cancer. | ✓
33% success with v1 model |
Tumor Necrosis Factor alpha (TNF-ɑ) | 1TNF | Cytokine that triggers inflammation and a treatment target for autoimmune disease. AlphaProteo failed to generate a binder for this target. | ✗
0% success with v1 and v2 models |
Key Takeaways and Implications
AlphaProteo is still closed source and has not been released to the community to test and use; third-party validation and peer review are just as important for software as it is for hardware testing. AlphaProteo, however, has amazing future use cases for improving medicinal and biomolecular practice.
Future applications can benefit from capabilities starting from sequence only, delivering agile protein design for accelerated therapeutic candidate generation. The faster we can narrow and find possibilities, the less work is needed to test a whole breadth of candidates—ideal for tackling evolving pathogens and fast-moving pandemics. The original AlphaFold was a pivotal player in uncovering the mysteries of SARS-CoV-2 or COVID-19.
We can also expect progress in precision protein regulatory targeting. Many protein therapeutics available today work by the crude method of binding a target through sheer agglomeration to reduce its activity. While these methods are effective for commercial drug companies and their successful products, nature has shown us much more sophisticated and precise means for regulating protein function. More sophisticated therapeutic biologics of the future may be able to precisely modulate target proteins and their activity, and AlphaProteo could be the start.
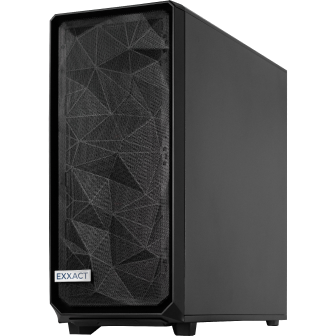
We're Here to Deliver the Tools to Power Your Research
With access to the highest performing hardware, at Exxact, we can offer the platform optimized for your deployment, budget, and desired performance so you can make an impact with your research!
Configure your Life Science Solution Today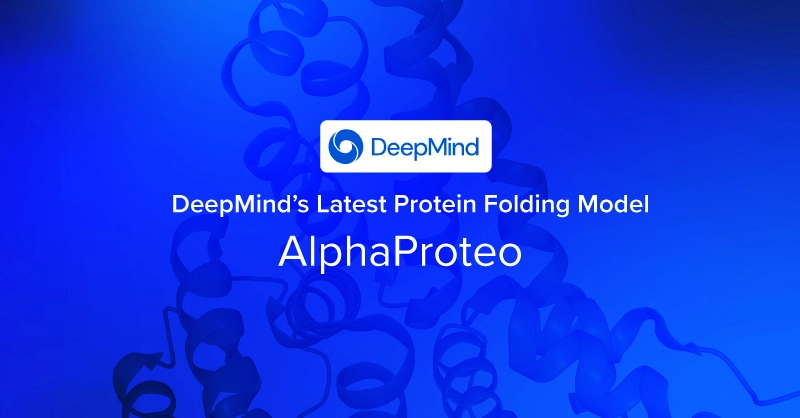
AlphaProteo - DeepMind’s Latest Protein Folding Model
Introduction
In 2020, DeepMind released their revolutionary technology AlphaFold, an AI-powered model for predicting the 3D structure of proteins based on their amino acid sequences. This breakthrough achieved unprecedented accuracy at 80% confidence, revolutionizing the life science industry and propelling research to new heights.
Since then, many research disciplines have incorporated AI protein folding algorithms into their workflows for generating novel designs, validating structural predictions, and testing interactions. Following the successful releases of AlphaFold2 and AlphaFold3, along with a public database containing over 200 million predicted protein structures, DeepMind and Google have introduced AlphaProteo—a model specifically engineered to predict protein binding.
AlphaProteo - Predicting Protein-to-Protein Binding
The AlphaFold group described AlphaProteo as a new system for generating protein-binding protein designs. Unlike previous offerings, AlphaProteo was developed specifically to model and design protein-protein interactions with an emphasis on binding from the start.
Unlike making use of an AlphaFold model plus clever tricks to predict binding (like a long flexible glycine linker connecting two protein sequences of interest), AlphaProteo builds models to deal with protein complexes with ligands, multiple chains, or short peptides.
Notably, it was designed specifically to enable protein engineers to specify a target protein and a specific epitope or residues present on a particular part of a folded protein's surface with regards to movement. These movements are crucial for understanding how proteins perform their functions in the body, such as catalyzing reactions, signaling between cells, or binding to specific molecules. Developing a protein binder designed to tightly target another protein is an extremely difficult problem but particularly useful for a wide range of applications.
AlphaProteo was trained on experimentally determined protein structures from the Protein Data Bank (PDB), as well as over 100 million predicted structures generated by AlphaFold. For evaluation, they focused on only eight targets. Most of these are of particular interest for their prominent role in human health and disease. The percentage represents the number of successful binders generated to bind to the target validated in a wet lab with cryo-EM and x-ray crystallography.
Name | PDB ID | Importance | Success? |
BHRF1 | 2WH6 | Oncogenic protein from Epstein-Barr virus; can slow or stop tumor growth. | ✓
88% success with v1 model |
SARS-CoV-2 spike protein (SC2RBD) | 6M0J | Involved in cell surface binding and invasion. Disrupting spike protein binding can block COVID infection. | ✓
29% success with v1 model and improved filters |
Interleukin-7 Receptor (IL-7RA) | 3DI3 | Cell surface receptor on lymphocytes, treatment target for some leukemias and HIV. | ✓
24.5% success with v1 model |
Interleukin-17A (IL-17A) | 4HSA | Secreted protein that triggers inflammation and target in treating autoimmune disease. | ✓
14.3% success with v1 model |
Tropomyosin Receptor Kinase A (TrkA) | 1WWW | Nerve growth factor involved in autoimmune disease and target in treating chronic pain | ✓
8.1% success with v2 model |
Programmed Death Ligand (PD-L1) | 5O45 | Cell surface receptor involved in regulating immune cell proliferation, target for treating some cancers. | ✓26.5% success rate with v2 model |
Vascular Endothelial Growth Factor A (VEGF-A) | 1BJ1 | Secreted growth factor that controls angiogenesis (blood vessel formation), e.g., used by cancer cells to recruit blood supply and a target in treating cancer. | ✓
33% success with v1 model |
Tumor Necrosis Factor alpha (TNF-ɑ) | 1TNF | Cytokine that triggers inflammation and a treatment target for autoimmune disease. AlphaProteo failed to generate a binder for this target. | ✗
0% success with v1 and v2 models |
Key Takeaways and Implications
AlphaProteo is still closed source and has not been released to the community to test and use; third-party validation and peer review are just as important for software as it is for hardware testing. AlphaProteo, however, has amazing future use cases for improving medicinal and biomolecular practice.
Future applications can benefit from capabilities starting from sequence only, delivering agile protein design for accelerated therapeutic candidate generation. The faster we can narrow and find possibilities, the less work is needed to test a whole breadth of candidates—ideal for tackling evolving pathogens and fast-moving pandemics. The original AlphaFold was a pivotal player in uncovering the mysteries of SARS-CoV-2 or COVID-19.
We can also expect progress in precision protein regulatory targeting. Many protein therapeutics available today work by the crude method of binding a target through sheer agglomeration to reduce its activity. While these methods are effective for commercial drug companies and their successful products, nature has shown us much more sophisticated and precise means for regulating protein function. More sophisticated therapeutic biologics of the future may be able to precisely modulate target proteins and their activity, and AlphaProteo could be the start.
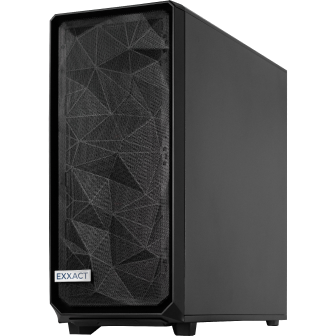
We're Here to Deliver the Tools to Power Your Research
With access to the highest performing hardware, at Exxact, we can offer the platform optimized for your deployment, budget, and desired performance so you can make an impact with your research!
Configure your Life Science Solution Today