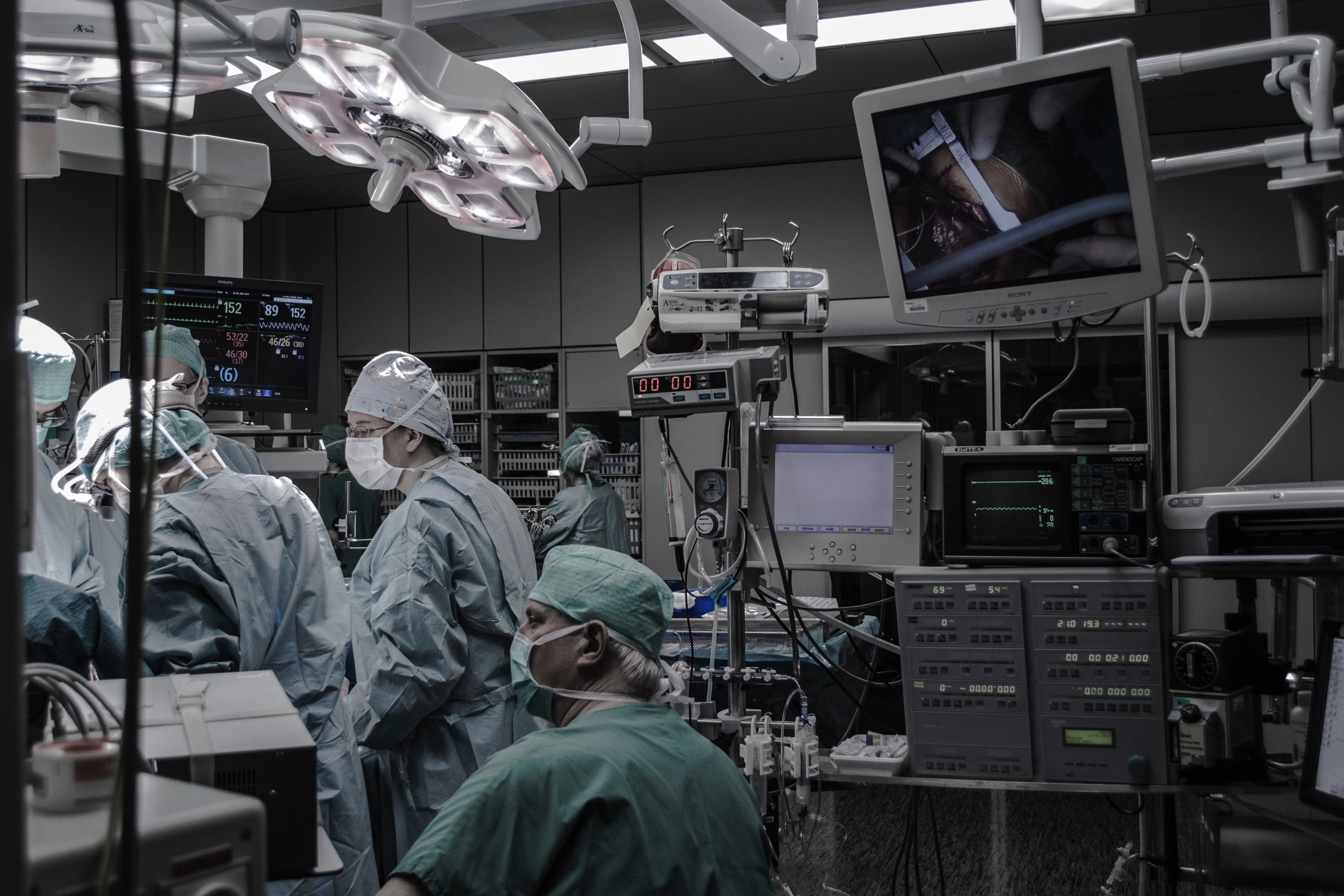
Research firm, Gartner, expects the global AI economy to increase from about $1.2 trillion last year to about $3.9 Trillion by 2022, while McKinsey sees it delivering global economic activity of around $13 trillion by 2030. And of course, this transformation is fueled by the powerful Machine Learning (ML) tools and techniques such as Deep Reinforcement Learning (DRL), Generative Adversarial Networks (GAN), Gradient-boosted-tree models (GBM), etc.
Along with commercial business and technology, healthcare is a field that is thought to be most suitable to be profoundly impacted by AI tools and techniques. Mandatory practices such as Electronic Medical Records (EMR) have already primed healthcare for the application of Big Data tools for advanced analytics. AI and ML hold significant promise to inject further value and enhance the degree of automation and the quality of intelligent decision-making in patient care and public health systems to transform the lives of billions around the world.
Machine Learning Applications in Healthcare
In this section, we will cite some key examples of the modern application of AI/ML techniques in healthcare settings. Some of these are specific to the problem being solved, others are more generic in nature.
Breast Cancer & Other Forms of Cancer Diagnosis
Did you know, close to two million new cancer cases are diagnosed every year in the U.S. and more than half a million deaths are reported from cancer? Breast cancer is listed as the top contributor to these cases. Deep learning (DL) and other classification and image processing techniques are being used in the fight against breast cancer (and other forms of cancers too).
MRI and other advanced medical imaging systems are being equipped with ML algorithms and they are increasingly forming the first line of checks for cancer detection. Often the number and quality of radiologists fall short in the face of overwhelming digitized data coming out of these imaging systems and ML-based systems are a perfect choice for aiding their decision-making process.
An article below has a comprehensive overview of various efforts in this regard.
Deep Learning & AI is Improving the Accuracy of Breast Cancer Detection
ML techniques are being used for other forms of cancer detection too. AI has been improving the accuracy of breast cancer detection. A recent article from Nature, for example, describes how ML is applied to perform low-level image analysis tasks such as prostate segmentation and fusion of different modalities (for example MRI, CT, and ultrasonography).
ML tools are also adding significant value by augmenting the surgeon’s display with information such as cancer localization during robotic procedures and other image-guided interventions.
AI & Machine Learning Assisting in Radiology & Pathology
The use of AI/ML techniques in assisting radiologists will only expand exponentially in the future. Throughout the world, and especially in the US, trained radiologists are under enormous strain due to the deluge of digital medical data. A Nature article estimates that the average radiologist needs to interpret one image every 3–4 seconds in an 8-hour workday to meet workload demands.
Imaging data is plentiful these days and DL algorithms can be fed with an ever-expanding dataset of medical images, to find patterns and interpret the results as a highly trained radiologist would. The algorithm can be trained to sort through regular and abnormal results, identifying suspicious spots on the skin, lesions, tumors, and brain bleeds, for example.
These kinds of ML methods can be particularly helpful in identifying rare or difficult to diagnose diseases as they are built on large datasets containing images of these diseases and they are often more dependable than humans when it comes to detecting ‘edge-cases’ (if trained properly).
For example, Microsoft's Project InnerEye employs machine learning to differentiate between tumors and healthy anatomy using 3D radiological images that assist medical experts in radiotherapy and surgical planning. In their own words, it can help in the following ways:
- extraction of targeted radiomics measurements for quantitative radiology,
- efficient contouring for radiotherapy planning,
- precise surgery planning and navigation.
Machine Learning & Data Science for Actionable Medical Insights
An ever-increasing amount of medical data are being digitized at all public and private healthcare institutions (hospitals, doctors’ clinics, pathology centers, etc.). However, by their very nature, this kind of data is messy and unstructured. Unlike other types of business data, where traditional statistical methods can be used for quick insights, patient data is not particularly amenable to simple modeling and analytics tools.
We need highly dependable and robust platforms that can connect to a multitude of patient databases and analyze a mixture of radiology images, blood tests, EKGs, genomics, patient medical history, etc. Furthermore, they should be able to distill down their analyses and the hidden patterns that they discover, to human-intelligible forms so that doctors and other healthcare professionals can work on their output to prescribe affordable and responsible patient care regimes.
For example, Enlitic, a San Francisco based start-up, has a mission of mixing intelligence with empathy and leverage the power of AI for precisely generating such insights, as discussed above. In their own words, “by pairing world-class radiologists with data scientists and engineers, we collect and analyze the world’s most comprehensive clinical data, pioneering medical software that enables doctors to diagnose sooner with renowned accuracy.”
AI for Healthcare Operation Management & Patient Experience
The cost and difficulty of receiving proper health care have been a subject of long and bitter debate in the US. Americans, on average, visits doctors only slightly above four times a year, which is much less compared to that in other developed nations (13 times for Japan, for example).
What are the pressing issues for avoiding healthcare systems? Long queue, fear of getting unreasonable bills, frustrating appointment process, not getting paired up with the right professional.
AI and associated data-driven techniques are uniquely poised to tackle those problems for rationalizing the operational issues in a healthcare system. Sometimes, the problem is that of an optimum staff allocation, in other times, it is finding a proper match between a patient and a professional. Traditional businesses have been facing such problems for many decades and AI and ML techniques have already found their way into the solution stack because such pattern matching or optimization problems are best solved using huge database and intelligent search algorithms which are a strength of AI tools.
As described in this article, patient engagement and adherence have long been seen as the ‘last mile’ problem of healthcare – the final barrier between ineffective and good health-related outcomes. The more patients proactively participate in their own well-being, the better the outcomes – resource utilization, financial outcomes, and stakeholder experience. These issues are increasingly being addressed by the unique and powerful combination of Big Data and AI.
Therefore, hospitals and public health organizations must leverage similar solutions for their day-to-day operational problems. What is most appealing is that for these particular problems, the concern of data privacy, which is a complex and difficult issue for healthcare systems, poses the least hindrance. This is because, often the operational problem does not involve confidential patient data related to disease, diagnosis, or medicine but is related to finance, capital infrastructure, marketing, or human resource issues of a large organization much like any other modern business enterprise.
The key here is to develop and employ suitable AI-assisted platforms whose primary mission is to enhance the experience of healthcare services for the largest section of common people. As mentioned above, traditional businesses in many sectors, have started large-scale deployment of such tools for improving their operational efficiency. But most often, the overarching goal of such platforms is to maximize profit for the businesses. The distinguishing feature for adopting similar powerful AI tools for healthcare organizations will be to carefully mix the aspects of empathy with the goal of profit generation.
Physical Robots & Robotic Process Automation in Healthcare
It is no secret that surgical robots provide a unique kind of ‘superpower’ to human surgeons, improving their ability to see and navigate, create precise and minimally invasive incisions, stitch wounds with precision and minimal pain, and so much more. Use of such robots was initially approved in the USA in 2000, and since then they have been growing in numbers and scope, used in all kinds of procedures such as orthopedics, urology, gynecology, neurology, thoracic, otolaryngology, bariatric, rectal, and colon, and even multiple oncologies.
What are the possibilities of AI and ML being used for surgical robotic assistants? A recent article from RoboticsBusinessReview has explored the following topics for surgical robotic assistants:
- Distributed data-processing and software-centric collaboration between a team of surgical robots
- AI-generated virtual reality space (built based on thousands of historical images and 3D data streams) which can direct and guide a surgeon real-time
- Data-driven insights and advice based on previous surgery histories (not only performed by machines but by humans)
- The increasing possibility of fusion with telemedicine and remote surgery for relatively simple procedures
Drug Discovery Aided by Artificial Intelligence (AI) & Machine Learning (ML) Techniques
U.S. pharma companies, on average, spend over 2.5 billion dollars per successful drug development and market launch. This stiff price tag, no doubt, impacts the overall cost of healthcare for the general population.
AI and ML techniques are increasingly being chosen by big names in the pharma industry to solve this hellishly difficult issue. AI and ML is very effective at hunting for new pharmaceutical opportunities in the industry in examples like:
- Sanofi has signed a deal to use UK start-up Exscientia’s AI platform to hunt for metabolic-disease therapies,
- Genentech is using an AI system from GNS Healthcare in Cambridge, Massachusetts, to help drive its search for cancer-related treatments,
- Pfizer is using IBM Watson platform and its vast array of analytics and ML engines, to power its search for immuno-oncology drugs.
Apart from the long-haul chain of drug discovery, fundamental processes of early-stage candidate selection and even mechanism discovery are being thought to be particularly amenable to AI techniques. For instance, researchers at the biotechnology company Berg are working on a model to identify previously unknown cancer mechanisms using tests on more than 1,000 cancerous and healthy human cell samples and tracking data from their lipid, metabolite, enzyme, and protein profiles. The group uses its AI platform to generate and analyze immense amounts of biological and outcomes data from patients to highlight key differences between diseased and healthy cells.
Increasingly, many start-up firms are feeding data from sources such as research papers, patents, clinical trials, and patient records into the AI system. Such systems utilize the latest techniques in Bayesian inference, Markov chain models, reinforcement learning, and natural language processing (NLP) to help analyze the data intelligently for finding patterns and constructing high-dimensional representations, to be stored in the cloud and used in the drug-discovery process.
Precision Medicine & Preventive Healthcare–the Ultimate Frontier for Artificial Intelligence (AI) & Machine Learning (ML)
The development of high-throughput, data-intensive biomedical research assays and application of large-scale statistical modeling to such datasets have already revealed a great heterogeneity in the pathophysiologic factors and processes that contribute to disease, elucidating the fact that there is a need to tailor or personalize, medicinal regimens to the nuanced and often unique features possessed by individual patients.
According to the U.S. National Library of Medicine, precision medicine is "an emerging approach for disease treatment and prevention that takes into account individual variability in genes, environment, and lifestyle for each person." And this is one of the biggest anticipated benefits from the application of AI and ML in healthcare.
Without the help of advanced AI and ML tools such as deep neural networks, AI-driven search algorithms, or probabilistic graph models, the prospect of finding precise treatment option for an individual based on his or her personal medical history, lifestyle choices, genetic database, and dynamically changing pathological tests, seems infeasible.
And, according to a recent Forbes article, the added advantage of such an AI-driven system will be the ability to not only predict and model outcomes, but also to predict future patients’ probability of having diseases given early screening or routine annual physical exam data. By being able to model why and in what environments diseases are more likely to occur, AI tools can prepare medical professionals to intervene (in a personalized manner) before a disease is showing symptoms.
We Are Just Beginning to Realize the Potential of Artificial Intelligence (AI) & Machine Learning (ML) in Healthcare
In this article, we discussed a wide variety of exciting applications of powerful AI/ML techniques and platforms, built on those algorithms, in the space of healthcare. And we also discussed topics ranging from pathological assistant to operations management, to personalized medicine, and drug discovery.
There are, of course, known challenges from data privacy and legal frameworks. Health and patient-related data are some of the most closely guarded secrets and it is extremely complex to figure out what can be used legally by private third-party tool providers (in this case the owner of the AI and ML tools or platforms).
Therefore, a massive parallel effort to rationalize the legal and policy-making is needed to bring the full benefit of advancement in AI technologies into the healthcare space. As technologists and AI/ML enthusiasts, we can only hope for such a bright future where the power of these intelligent algorithms benefit millions of common people to improve their well-being.
xtagstartz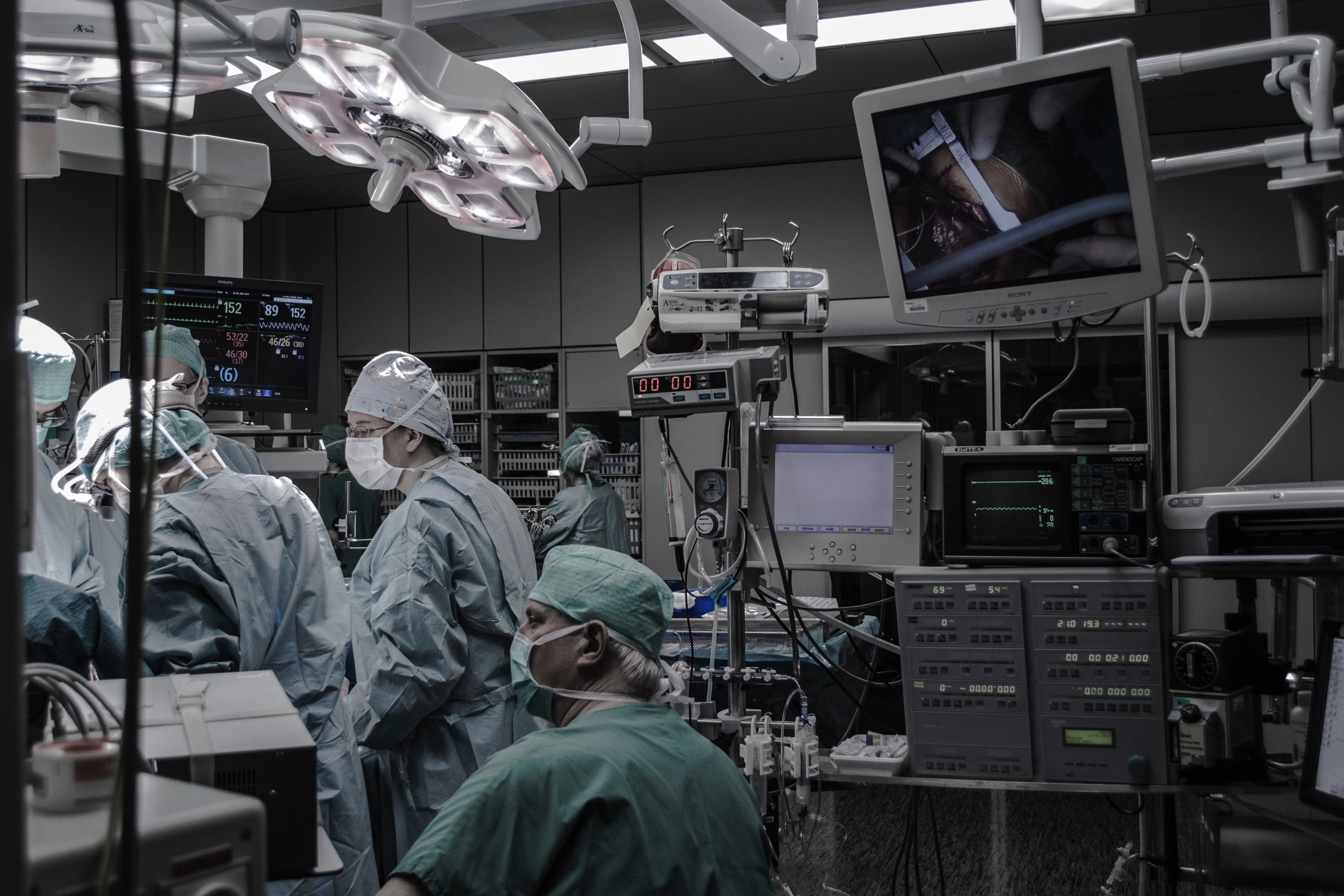
Artificial Intelligence (AI) & Machine Learning (ML) in Healthcare: Examples of Its Potential in Medicine
Research firm, Gartner, expects the global AI economy to increase from about $1.2 trillion last year to about $3.9 Trillion by 2022, while McKinsey sees it delivering global economic activity of around $13 trillion by 2030. And of course, this transformation is fueled by the powerful Machine Learning (ML) tools and techniques such as Deep Reinforcement Learning (DRL), Generative Adversarial Networks (GAN), Gradient-boosted-tree models (GBM), etc.
Along with commercial business and technology, healthcare is a field that is thought to be most suitable to be profoundly impacted by AI tools and techniques. Mandatory practices such as Electronic Medical Records (EMR) have already primed healthcare for the application of Big Data tools for advanced analytics. AI and ML hold significant promise to inject further value and enhance the degree of automation and the quality of intelligent decision-making in patient care and public health systems to transform the lives of billions around the world.
Machine Learning Applications in Healthcare
In this section, we will cite some key examples of the modern application of AI/ML techniques in healthcare settings. Some of these are specific to the problem being solved, others are more generic in nature.
Breast Cancer & Other Forms of Cancer Diagnosis
Did you know, close to two million new cancer cases are diagnosed every year in the U.S. and more than half a million deaths are reported from cancer? Breast cancer is listed as the top contributor to these cases. Deep learning (DL) and other classification and image processing techniques are being used in the fight against breast cancer (and other forms of cancers too).
MRI and other advanced medical imaging systems are being equipped with ML algorithms and they are increasingly forming the first line of checks for cancer detection. Often the number and quality of radiologists fall short in the face of overwhelming digitized data coming out of these imaging systems and ML-based systems are a perfect choice for aiding their decision-making process.
An article below has a comprehensive overview of various efforts in this regard.
Deep Learning & AI is Improving the Accuracy of Breast Cancer Detection
ML techniques are being used for other forms of cancer detection too. AI has been improving the accuracy of breast cancer detection. A recent article from Nature, for example, describes how ML is applied to perform low-level image analysis tasks such as prostate segmentation and fusion of different modalities (for example MRI, CT, and ultrasonography).
ML tools are also adding significant value by augmenting the surgeon’s display with information such as cancer localization during robotic procedures and other image-guided interventions.
AI & Machine Learning Assisting in Radiology & Pathology
The use of AI/ML techniques in assisting radiologists will only expand exponentially in the future. Throughout the world, and especially in the US, trained radiologists are under enormous strain due to the deluge of digital medical data. A Nature article estimates that the average radiologist needs to interpret one image every 3–4 seconds in an 8-hour workday to meet workload demands.
Imaging data is plentiful these days and DL algorithms can be fed with an ever-expanding dataset of medical images, to find patterns and interpret the results as a highly trained radiologist would. The algorithm can be trained to sort through regular and abnormal results, identifying suspicious spots on the skin, lesions, tumors, and brain bleeds, for example.
These kinds of ML methods can be particularly helpful in identifying rare or difficult to diagnose diseases as they are built on large datasets containing images of these diseases and they are often more dependable than humans when it comes to detecting ‘edge-cases’ (if trained properly).
For example, Microsoft's Project InnerEye employs machine learning to differentiate between tumors and healthy anatomy using 3D radiological images that assist medical experts in radiotherapy and surgical planning. In their own words, it can help in the following ways:
- extraction of targeted radiomics measurements for quantitative radiology,
- efficient contouring for radiotherapy planning,
- precise surgery planning and navigation.
Machine Learning & Data Science for Actionable Medical Insights
An ever-increasing amount of medical data are being digitized at all public and private healthcare institutions (hospitals, doctors’ clinics, pathology centers, etc.). However, by their very nature, this kind of data is messy and unstructured. Unlike other types of business data, where traditional statistical methods can be used for quick insights, patient data is not particularly amenable to simple modeling and analytics tools.
We need highly dependable and robust platforms that can connect to a multitude of patient databases and analyze a mixture of radiology images, blood tests, EKGs, genomics, patient medical history, etc. Furthermore, they should be able to distill down their analyses and the hidden patterns that they discover, to human-intelligible forms so that doctors and other healthcare professionals can work on their output to prescribe affordable and responsible patient care regimes.
For example, Enlitic, a San Francisco based start-up, has a mission of mixing intelligence with empathy and leverage the power of AI for precisely generating such insights, as discussed above. In their own words, “by pairing world-class radiologists with data scientists and engineers, we collect and analyze the world’s most comprehensive clinical data, pioneering medical software that enables doctors to diagnose sooner with renowned accuracy.”
AI for Healthcare Operation Management & Patient Experience
The cost and difficulty of receiving proper health care have been a subject of long and bitter debate in the US. Americans, on average, visits doctors only slightly above four times a year, which is much less compared to that in other developed nations (13 times for Japan, for example).
What are the pressing issues for avoiding healthcare systems? Long queue, fear of getting unreasonable bills, frustrating appointment process, not getting paired up with the right professional.
AI and associated data-driven techniques are uniquely poised to tackle those problems for rationalizing the operational issues in a healthcare system. Sometimes, the problem is that of an optimum staff allocation, in other times, it is finding a proper match between a patient and a professional. Traditional businesses have been facing such problems for many decades and AI and ML techniques have already found their way into the solution stack because such pattern matching or optimization problems are best solved using huge database and intelligent search algorithms which are a strength of AI tools.
As described in this article, patient engagement and adherence have long been seen as the ‘last mile’ problem of healthcare – the final barrier between ineffective and good health-related outcomes. The more patients proactively participate in their own well-being, the better the outcomes – resource utilization, financial outcomes, and stakeholder experience. These issues are increasingly being addressed by the unique and powerful combination of Big Data and AI.
Therefore, hospitals and public health organizations must leverage similar solutions for their day-to-day operational problems. What is most appealing is that for these particular problems, the concern of data privacy, which is a complex and difficult issue for healthcare systems, poses the least hindrance. This is because, often the operational problem does not involve confidential patient data related to disease, diagnosis, or medicine but is related to finance, capital infrastructure, marketing, or human resource issues of a large organization much like any other modern business enterprise.
The key here is to develop and employ suitable AI-assisted platforms whose primary mission is to enhance the experience of healthcare services for the largest section of common people. As mentioned above, traditional businesses in many sectors, have started large-scale deployment of such tools for improving their operational efficiency. But most often, the overarching goal of such platforms is to maximize profit for the businesses. The distinguishing feature for adopting similar powerful AI tools for healthcare organizations will be to carefully mix the aspects of empathy with the goal of profit generation.
Physical Robots & Robotic Process Automation in Healthcare
It is no secret that surgical robots provide a unique kind of ‘superpower’ to human surgeons, improving their ability to see and navigate, create precise and minimally invasive incisions, stitch wounds with precision and minimal pain, and so much more. Use of such robots was initially approved in the USA in 2000, and since then they have been growing in numbers and scope, used in all kinds of procedures such as orthopedics, urology, gynecology, neurology, thoracic, otolaryngology, bariatric, rectal, and colon, and even multiple oncologies.
What are the possibilities of AI and ML being used for surgical robotic assistants? A recent article from RoboticsBusinessReview has explored the following topics for surgical robotic assistants:
- Distributed data-processing and software-centric collaboration between a team of surgical robots
- AI-generated virtual reality space (built based on thousands of historical images and 3D data streams) which can direct and guide a surgeon real-time
- Data-driven insights and advice based on previous surgery histories (not only performed by machines but by humans)
- The increasing possibility of fusion with telemedicine and remote surgery for relatively simple procedures
Drug Discovery Aided by Artificial Intelligence (AI) & Machine Learning (ML) Techniques
U.S. pharma companies, on average, spend over 2.5 billion dollars per successful drug development and market launch. This stiff price tag, no doubt, impacts the overall cost of healthcare for the general population.
AI and ML techniques are increasingly being chosen by big names in the pharma industry to solve this hellishly difficult issue. AI and ML is very effective at hunting for new pharmaceutical opportunities in the industry in examples like:
- Sanofi has signed a deal to use UK start-up Exscientia’s AI platform to hunt for metabolic-disease therapies,
- Genentech is using an AI system from GNS Healthcare in Cambridge, Massachusetts, to help drive its search for cancer-related treatments,
- Pfizer is using IBM Watson platform and its vast array of analytics and ML engines, to power its search for immuno-oncology drugs.
Apart from the long-haul chain of drug discovery, fundamental processes of early-stage candidate selection and even mechanism discovery are being thought to be particularly amenable to AI techniques. For instance, researchers at the biotechnology company Berg are working on a model to identify previously unknown cancer mechanisms using tests on more than 1,000 cancerous and healthy human cell samples and tracking data from their lipid, metabolite, enzyme, and protein profiles. The group uses its AI platform to generate and analyze immense amounts of biological and outcomes data from patients to highlight key differences between diseased and healthy cells.
Increasingly, many start-up firms are feeding data from sources such as research papers, patents, clinical trials, and patient records into the AI system. Such systems utilize the latest techniques in Bayesian inference, Markov chain models, reinforcement learning, and natural language processing (NLP) to help analyze the data intelligently for finding patterns and constructing high-dimensional representations, to be stored in the cloud and used in the drug-discovery process.
Precision Medicine & Preventive Healthcare–the Ultimate Frontier for Artificial Intelligence (AI) & Machine Learning (ML)
The development of high-throughput, data-intensive biomedical research assays and application of large-scale statistical modeling to such datasets have already revealed a great heterogeneity in the pathophysiologic factors and processes that contribute to disease, elucidating the fact that there is a need to tailor or personalize, medicinal regimens to the nuanced and often unique features possessed by individual patients.
According to the U.S. National Library of Medicine, precision medicine is "an emerging approach for disease treatment and prevention that takes into account individual variability in genes, environment, and lifestyle for each person." And this is one of the biggest anticipated benefits from the application of AI and ML in healthcare.
Without the help of advanced AI and ML tools such as deep neural networks, AI-driven search algorithms, or probabilistic graph models, the prospect of finding precise treatment option for an individual based on his or her personal medical history, lifestyle choices, genetic database, and dynamically changing pathological tests, seems infeasible.
And, according to a recent Forbes article, the added advantage of such an AI-driven system will be the ability to not only predict and model outcomes, but also to predict future patients’ probability of having diseases given early screening or routine annual physical exam data. By being able to model why and in what environments diseases are more likely to occur, AI tools can prepare medical professionals to intervene (in a personalized manner) before a disease is showing symptoms.
We Are Just Beginning to Realize the Potential of Artificial Intelligence (AI) & Machine Learning (ML) in Healthcare
In this article, we discussed a wide variety of exciting applications of powerful AI/ML techniques and platforms, built on those algorithms, in the space of healthcare. And we also discussed topics ranging from pathological assistant to operations management, to personalized medicine, and drug discovery.
There are, of course, known challenges from data privacy and legal frameworks. Health and patient-related data are some of the most closely guarded secrets and it is extremely complex to figure out what can be used legally by private third-party tool providers (in this case the owner of the AI and ML tools or platforms).
Therefore, a massive parallel effort to rationalize the legal and policy-making is needed to bring the full benefit of advancement in AI technologies into the healthcare space. As technologists and AI/ML enthusiasts, we can only hope for such a bright future where the power of these intelligent algorithms benefit millions of common people to improve their well-being.
xtagstartz