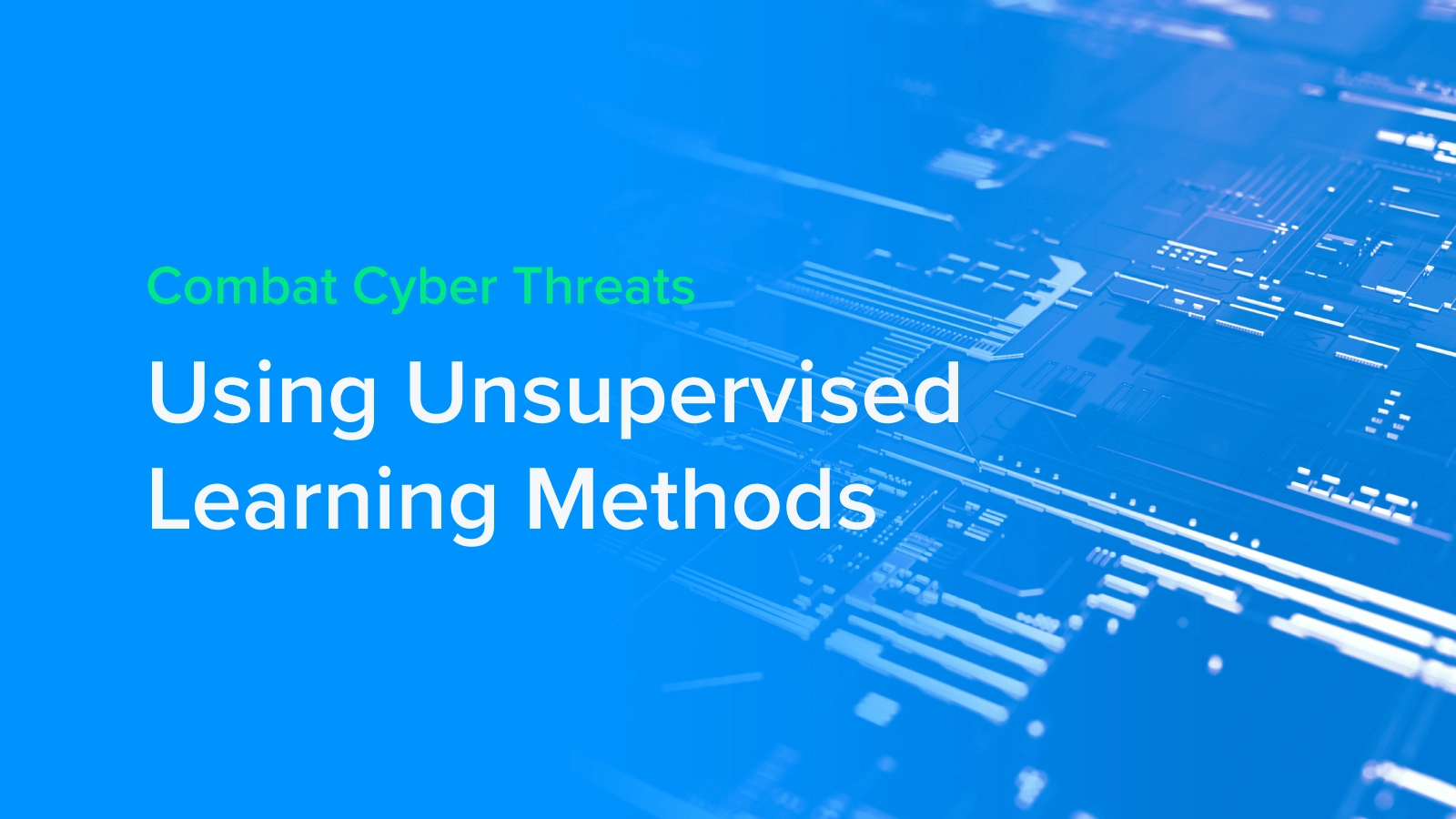
How Unsupervised Learning Methods Are Used to Combat Cyber Threats
As the world enters a fully digital age, cyber threats are on the rise with massive data breaches, hacks into personal and financial data, and any other digital source that people can exploit. To combat these attacks, security experts are increasingly tapping into AI to stay a step ahead using every tool in their toolbox including unsupervised learning methods.
Machine learning in the cybersecurity space is considered to still be in its infancy stage, but there has been a lot of traction since 2020 to have more AI involved in the process of combating cyber threats.
Understanding how machine learning can be used in cyber security, recognizing the need for unsupervised learning methods in cyber security, and knowing how to implement AI in combating cyber attacks are the key to fighting cybercrime in the years ahead.
Interested in an AI workstation?
Accelerate your machine learning research with an Exxact solution starting around $5,500
Using Machine Learning in Cybersecurity
The scary thing about cybercrime is that it can take up to six months to even detect a breach, and it takes an average of roughly 50 days from the time a breach is found to the time it is reported. That is a lot of time to be at the mercy of a cyber attack.
Machine learning can be used as a preventative measure to avoid cyber attacks. For example, cybersecurity systems can use machine learning to evaluate patterns (even in real-time!), learning from them in order to help prevent repeat assaults and respond to strange or changing behaviors.
Furthermore, it can assist cybersecurity teams in being more proactive in preventing threats and responding to live attacks rather than trying to pick up the pieces after the breach is discovered.
Unsupervised learning methods can actually begin to recognize patterns that may seem benign but are outside of the norm. These types of unusual behaviors can be easy to overlook in the regular tasks those in cyber security perform every day. It can help firm up their resources more strategically by reducing the amount of time spent on manual tasks.
Why You Need Unsupervised Learning Methods in Cybersecurity
When it comes to machine learning models, there are a variety of ways these models can be trained. There are supervised learning and unsupervised learning methods for the models, and it is the latter of these two that we will be discussing for the purposes of this article.
Unsupervised learning is a machine learning technique that does exactly what it's called: users do not need to supervise the model. Instead, the unsupervised model works on its own to discover patterns and information that was previously undetected.
In a nutshell: unsupervised learning methods for machine learning means there is little-to-no hands-on "training" of the AI model.
On the face of it, this may seem counterintuitive. Don’t you want to be able to train your machine learning model on how to recognize, identify, and report potential cyber attacks? Yes, but the problem is that there are so many ways a cyber criminal could choose to attack your organization or business that you may unintentionally train it to ignore other cyber threats.
Unsupervised learning allows for the AI models to come to their own conclusions in ways we might overlook. More importantly, it means you don’t have to experience a cyber attack or create a false scenario for your AI model to learn from!
This means unsupervised learning methods are able to forecast and protect against future threats without ever having to undergo a similar breach or attack.
Unsupervised learning involves processes known as clustering, representation learning, and density estimation. This allows these models to identify and group activities that may seem unusual, suspicious, or are at least unrecognized by the model and alert cybersecurity teams to what it may consider potential cyber threats.
Traditional cyber defense methods rely on data labeling to identify a particular danger and then implement a response. This can cause a delay that might be dangerous to a business and its digital assets.
How to Implement Unsupervised Learning Methods to Combat Cyber Threats
You never want to become too reliant on AI for your cybersecurity needs, but unsupervised learning models can be an asset to fighting these cyber attacks. Getting started, though, can feel a bit daunting.
The following steps can help you start training and implementing your unsupervised machine learning models on the right foot!
1. Identify Processes Where You Can Implement AI
Not all of the processes in cybersecurity are a good fit for a machine learning model. For example, a machine learning model trained by means of an unsupervised learning method would not be entirely helpful in correcting a data breach, but is excellent when helping catch the early signs of cyber attack attempts.
Carefully review your current cybersecurity strategies and processes to determine where AI models can be used and implemented well without taking away from the work of your cybersecurity team.
2. Establish Success Benchmarks for Your Unsupervised Learning Methods
Before letting your AI model run loose, there will need to be some benchmarks set. This will help you know that your machine learning model is actually helping and not hindering your cybersecurity efforts.
This also means that you will need to be creating a process for checking in with your AI model to ensure it is interpreting data correctly. When you know what success looks like for your unsupervised learning methods, then you will be able to course-correct appropriately.
3. Monitor & Report
Once your machine learning model is being trained on how to combat cyber threats and detect cyber attacks, monitoring and reporting are going to be critical steps for the success of your cybersecurity efforts.
Unsupervised learning methods can be powerful and effective, but these models can still interpret data incorrectly.
AI models that have been trained using unsupervised learning methods have been known to categorize and cluster data that, in all actuality, has nothing in common, so there has to be a process for correcting these interpretations as they arise.
For the most part, though, it is important to keep a close eye on these machine learning models simply because you never know when a human can begin to recognize cyber threats based on the data before the AI model can put the pieces together. Human intuition is still a huge part of cybersecurity that cannot be replicated by an AI.
Want to Learn More About AI and Cybersecurity?
Cyber threats are on the rise and unsupervised learning methods can help you create machine learning models for your cybersecurity teams that can combat cyber attacks.
Is there anything we didn’t cover here that you wanted to know more about? Is there some critical information you think we overlooked? We would love to hear your feedback and answer any questions or concerns you have about AI and cybersecurity. Just comment below or contact us to answer any questions.
Have any questions?
Contact Exxact Today
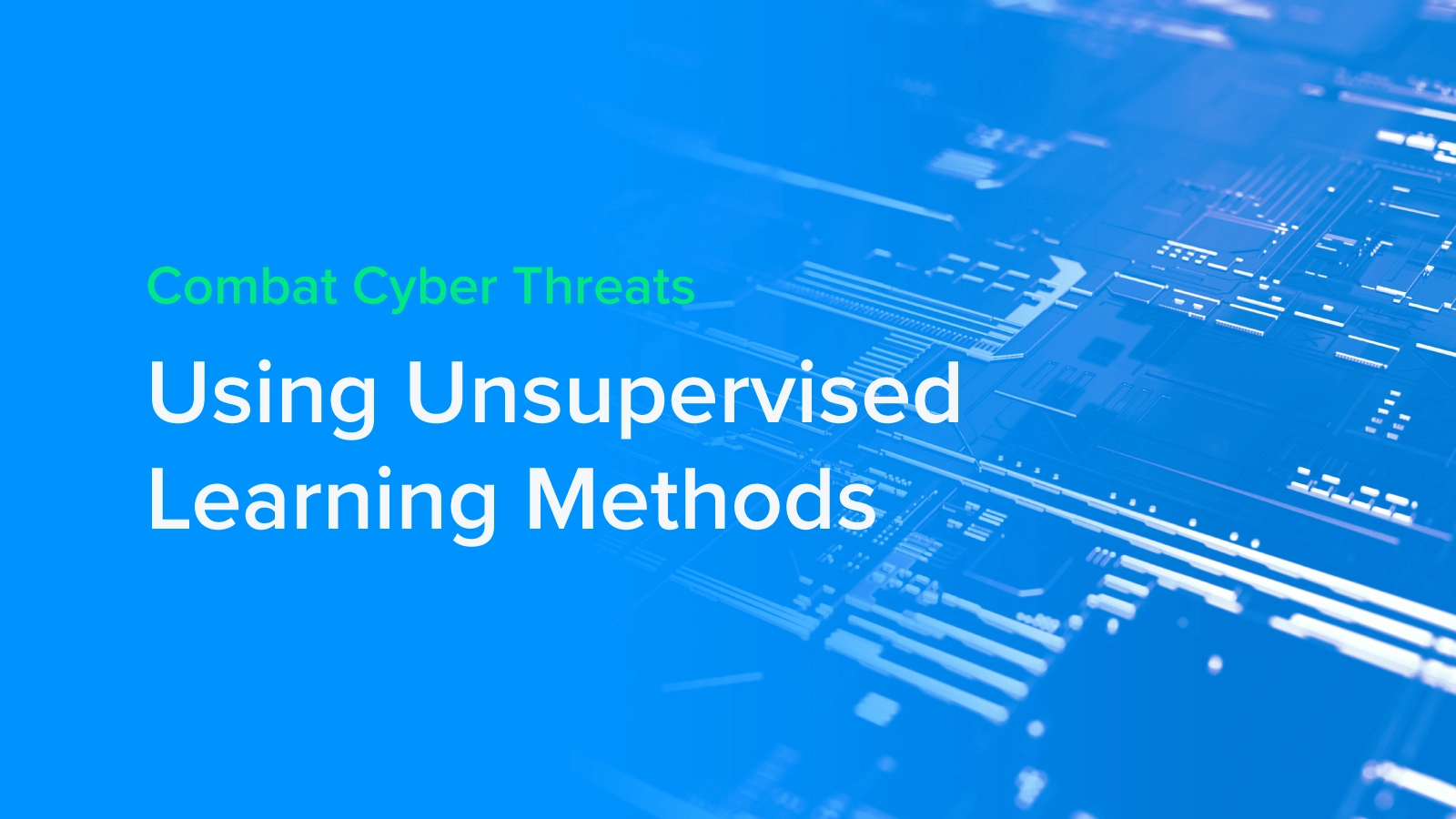
Using Unsupervised Learning to Combat Cyber Threats
How Unsupervised Learning Methods Are Used to Combat Cyber Threats
As the world enters a fully digital age, cyber threats are on the rise with massive data breaches, hacks into personal and financial data, and any other digital source that people can exploit. To combat these attacks, security experts are increasingly tapping into AI to stay a step ahead using every tool in their toolbox including unsupervised learning methods.
Machine learning in the cybersecurity space is considered to still be in its infancy stage, but there has been a lot of traction since 2020 to have more AI involved in the process of combating cyber threats.
Understanding how machine learning can be used in cyber security, recognizing the need for unsupervised learning methods in cyber security, and knowing how to implement AI in combating cyber attacks are the key to fighting cybercrime in the years ahead.
Interested in an AI workstation?
Accelerate your machine learning research with an Exxact solution starting around $5,500
Using Machine Learning in Cybersecurity
The scary thing about cybercrime is that it can take up to six months to even detect a breach, and it takes an average of roughly 50 days from the time a breach is found to the time it is reported. That is a lot of time to be at the mercy of a cyber attack.
Machine learning can be used as a preventative measure to avoid cyber attacks. For example, cybersecurity systems can use machine learning to evaluate patterns (even in real-time!), learning from them in order to help prevent repeat assaults and respond to strange or changing behaviors.
Furthermore, it can assist cybersecurity teams in being more proactive in preventing threats and responding to live attacks rather than trying to pick up the pieces after the breach is discovered.
Unsupervised learning methods can actually begin to recognize patterns that may seem benign but are outside of the norm. These types of unusual behaviors can be easy to overlook in the regular tasks those in cyber security perform every day. It can help firm up their resources more strategically by reducing the amount of time spent on manual tasks.
Why You Need Unsupervised Learning Methods in Cybersecurity
When it comes to machine learning models, there are a variety of ways these models can be trained. There are supervised learning and unsupervised learning methods for the models, and it is the latter of these two that we will be discussing for the purposes of this article.
Unsupervised learning is a machine learning technique that does exactly what it's called: users do not need to supervise the model. Instead, the unsupervised model works on its own to discover patterns and information that was previously undetected.
In a nutshell: unsupervised learning methods for machine learning means there is little-to-no hands-on "training" of the AI model.
On the face of it, this may seem counterintuitive. Don’t you want to be able to train your machine learning model on how to recognize, identify, and report potential cyber attacks? Yes, but the problem is that there are so many ways a cyber criminal could choose to attack your organization or business that you may unintentionally train it to ignore other cyber threats.
Unsupervised learning allows for the AI models to come to their own conclusions in ways we might overlook. More importantly, it means you don’t have to experience a cyber attack or create a false scenario for your AI model to learn from!
This means unsupervised learning methods are able to forecast and protect against future threats without ever having to undergo a similar breach or attack.
Unsupervised learning involves processes known as clustering, representation learning, and density estimation. This allows these models to identify and group activities that may seem unusual, suspicious, or are at least unrecognized by the model and alert cybersecurity teams to what it may consider potential cyber threats.
Traditional cyber defense methods rely on data labeling to identify a particular danger and then implement a response. This can cause a delay that might be dangerous to a business and its digital assets.
How to Implement Unsupervised Learning Methods to Combat Cyber Threats
You never want to become too reliant on AI for your cybersecurity needs, but unsupervised learning models can be an asset to fighting these cyber attacks. Getting started, though, can feel a bit daunting.
The following steps can help you start training and implementing your unsupervised machine learning models on the right foot!
1. Identify Processes Where You Can Implement AI
Not all of the processes in cybersecurity are a good fit for a machine learning model. For example, a machine learning model trained by means of an unsupervised learning method would not be entirely helpful in correcting a data breach, but is excellent when helping catch the early signs of cyber attack attempts.
Carefully review your current cybersecurity strategies and processes to determine where AI models can be used and implemented well without taking away from the work of your cybersecurity team.
2. Establish Success Benchmarks for Your Unsupervised Learning Methods
Before letting your AI model run loose, there will need to be some benchmarks set. This will help you know that your machine learning model is actually helping and not hindering your cybersecurity efforts.
This also means that you will need to be creating a process for checking in with your AI model to ensure it is interpreting data correctly. When you know what success looks like for your unsupervised learning methods, then you will be able to course-correct appropriately.
3. Monitor & Report
Once your machine learning model is being trained on how to combat cyber threats and detect cyber attacks, monitoring and reporting are going to be critical steps for the success of your cybersecurity efforts.
Unsupervised learning methods can be powerful and effective, but these models can still interpret data incorrectly.
AI models that have been trained using unsupervised learning methods have been known to categorize and cluster data that, in all actuality, has nothing in common, so there has to be a process for correcting these interpretations as they arise.
For the most part, though, it is important to keep a close eye on these machine learning models simply because you never know when a human can begin to recognize cyber threats based on the data before the AI model can put the pieces together. Human intuition is still a huge part of cybersecurity that cannot be replicated by an AI.
Want to Learn More About AI and Cybersecurity?
Cyber threats are on the rise and unsupervised learning methods can help you create machine learning models for your cybersecurity teams that can combat cyber attacks.
Is there anything we didn’t cover here that you wanted to know more about? Is there some critical information you think we overlooked? We would love to hear your feedback and answer any questions or concerns you have about AI and cybersecurity. Just comment below or contact us to answer any questions.
Have any questions?
Contact Exxact Today