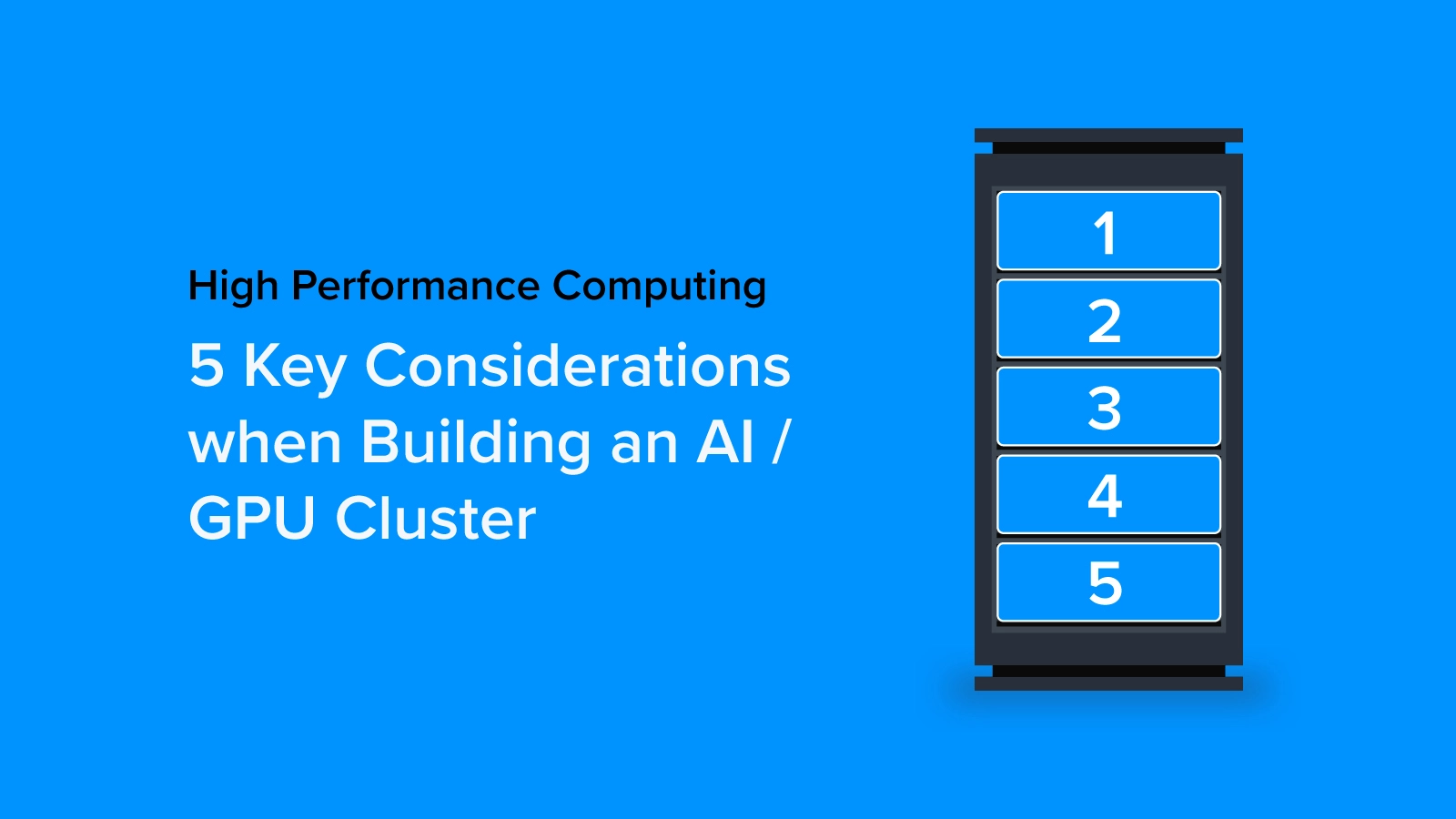
As deep learning applications are constantly evolving and developing, organizations are adapting to new technologies to increase performance and capabilities. Artificial intelligence is continuing to advance the way many organizations conduct their work and research. Failing to adapt and incorporate data science technologies runs the risk of falling behind the competition.
We at Exxact want to make AI and GPU Clusters accessible and easy to implement to propel your organization to its highest potential. There is a lot going on in the world of artificial intelligence and even more to think about when building a GPU-heavy AI workstation, server, or cluster system.
GPU Clusters General Use Cases
GPUs as you may know, provide the computational power and throughput to build, train, and deploy AI models. They collect data, generate new data, analyze existing data, automate tasks, and even enhance the way we interact with the world.
Almost every industry is being impacted by the use of AI such as:
- Shopping and Advertising
- Search Engines
- Digital Personal Assistants
- Translation
- Autonomous Vehicles and Devices
- Cybersecurity
- Life Science and Healthcare
- Transportation
- Industrial Manufacturing and Automation
- Food and Farming
With such an impact on the world’s most important industries, building a GPU cluster to help develop and conceptualize an AI model to accelerate your workloads is essential to propelling your business into modernism.
5 Essentials of an AI/GPU Cluster Infrastructure
1. Applications and Industry
The types of applications you plan to run will have a significant role in how you decide to build out your system. Deep learning made large advancements being brought into the life sciences and engineering field in recent years. Be sure to consider the applications you will need to be supported in your system when you spec out the perfect artificial intelligence system. Our engineers at Exxact can help you through this critical step and the unique needs of your organization.
Machine learning (ML) has evolved quite significantly over the past decade and has ramped up over the last few years. Machine learning is the study of AI where a model learns by analyzing patterns in a given dataset with no explicit programming. Machine learning applications can help organizations solve a wide range of problems, from science to engineering. This is done through the application of trained deep neural networks and complex data science algorithms which GPUs made feasible with parallel computation.
As deep learning plays an increasingly important role in our world’s organizations, it will become more and more important day over day to consider how these technological advancements will change the field of our work and how your organization can start leveraging its power.
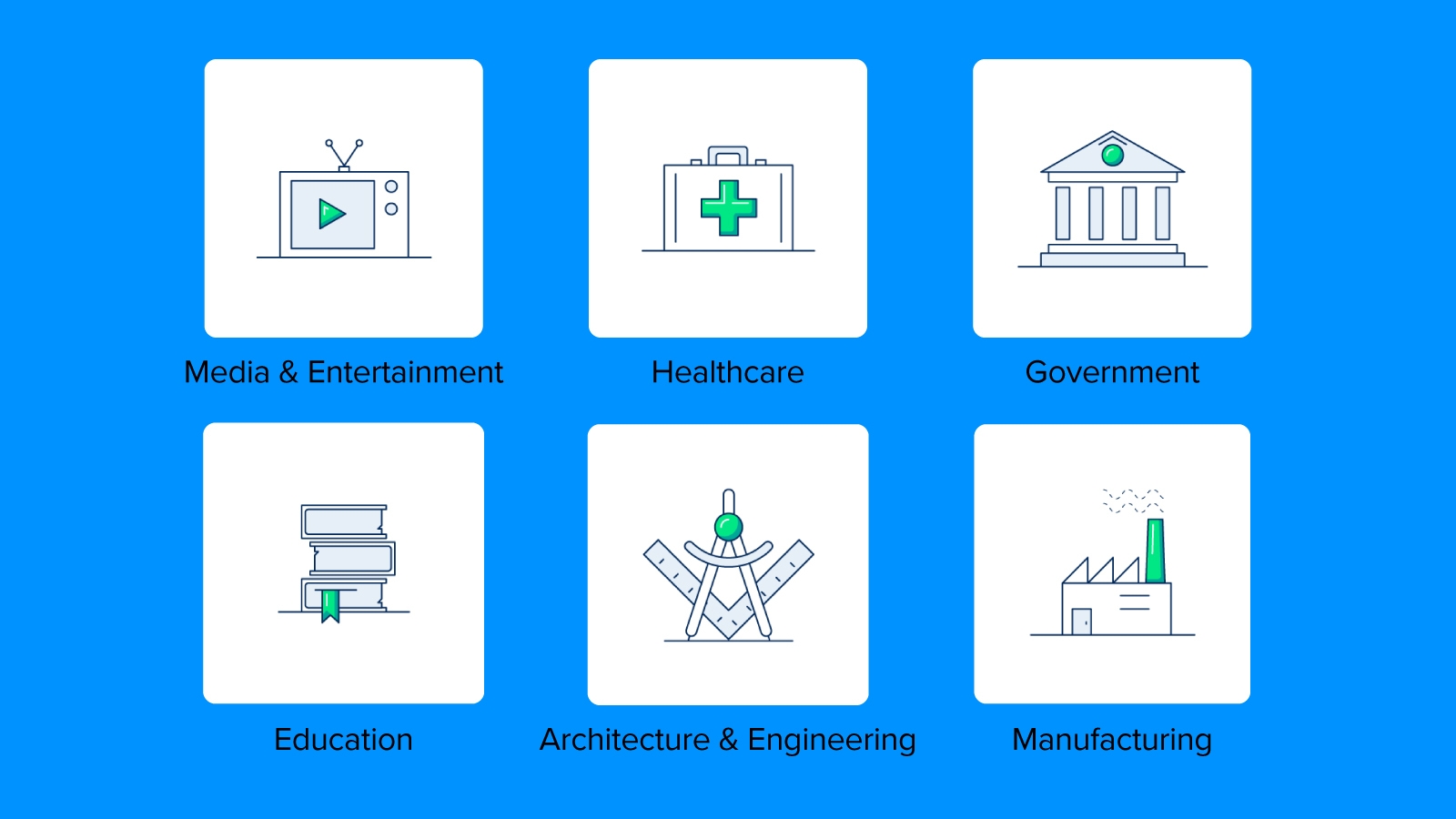
2. GPU Needs and Capabilities
When it comes to the right GPU selection, there are often so many choices to consider. Those starting out with Deep Learning and AI can get by using a competent gaming machine with a 3090 or 3090 Ti. You can also use Cloud Computing but it limits your capabilities and flexibility.
Among the most impressive GPU options is the NVIDIA A100, NVIDIA’s dedicated AI accelerator GPU built for speed and performance for scientific computing, graphics, and data analytics in data centers. NVIDIA’s next-generation NVIDIA H100 is coming to data centers in early 2023 which is claimed to have a 6x performance bump. The NVIDIA DGX Systems are the flagship and representation of peak AI computing.
The A100 and H100 are highly priced accordingly to their high efficiency, but if you’re not looking to break the bank, NVIDIA’s professional RTX lineup is a great alternative. Consider the RTX A6000, RTX A5500, or the new RTX 6000 Ada for your build.
However, if you are looking to delve straight in and need a competent machine, data center GPUs might be your best bet to save both on space, as well as increase performance per node. If you're not sure, you can always ask us!
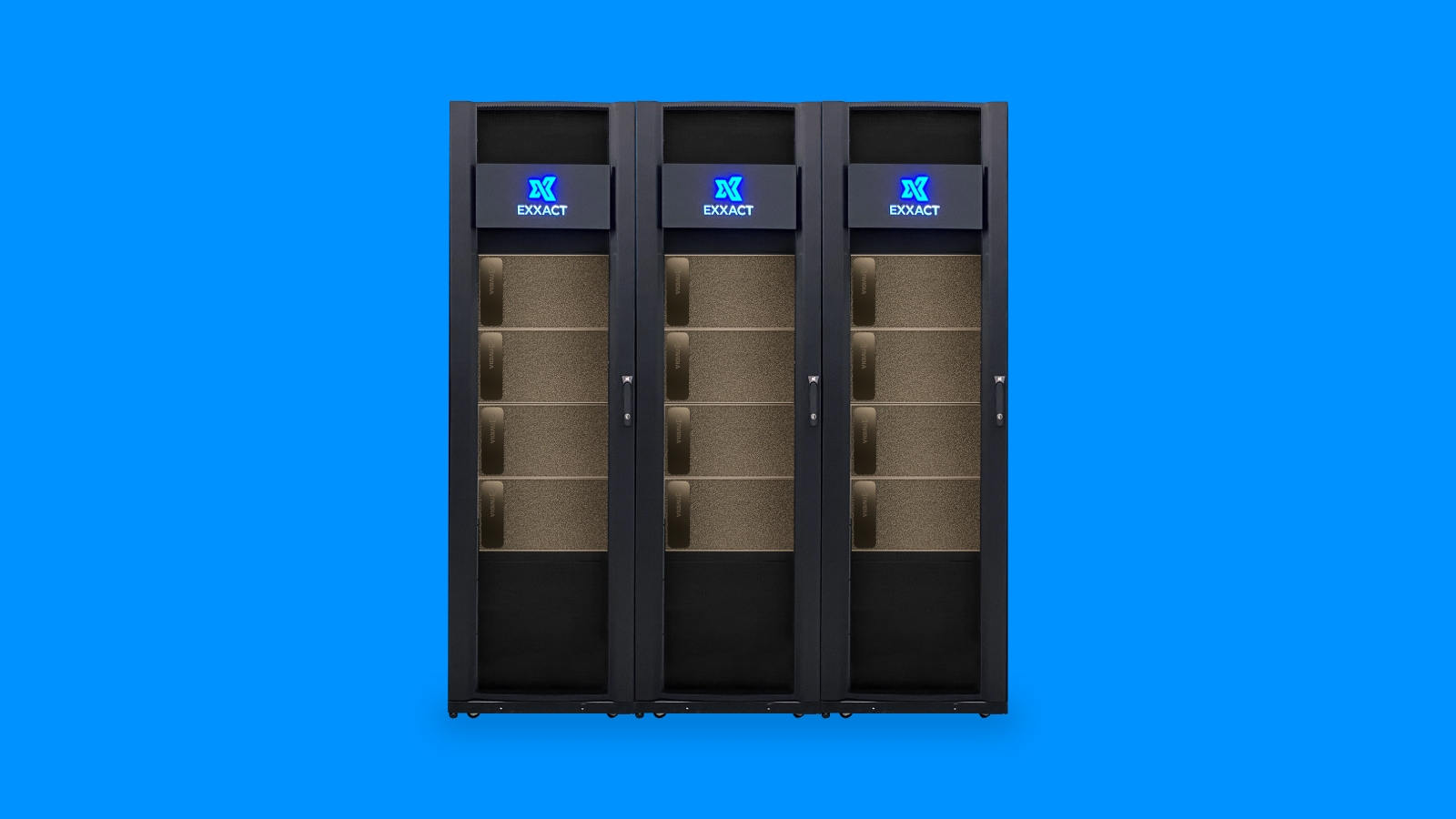
3. HPC Cluster vs. Single Server
We were hinting at it in the previous section. Consider whether you’ll need a single AI workstation a multi-GPU server or a large-scale HPC Cluster. This determination will often come down to budget constraints and the amount of data you plan to ingest, store, analyze, and process. AI/HPC server platforms offer a simple way for you to take control of your AI computing projects with maximum performance at the lowest total cost of ownership; sometimes a cluster is not always necessary when workloads are on a smaller scale.
Like the individual AI solutions (workstations and servers), our clusters come application-optimized with popular industry applications. Check out Exxact’s Supported Software page here, or ask our engineers about your specific program and we can do our best to inform you of capabilities and recommended solutions.
4. AI Infrastructure Needs
For large GPU systems, power and cooling are the main concerns. AI servers draw significantly more power than previous-generation CPU-only servers, with some of the higher-end platforms maxing out at 6000 watts. Ensuring that your facility can provide adequate power is essential in determining the size and breadth of your system.
An HVAC unit and environment are necessary to remove the heat created by these systems in operation. These GPU clusters need to be properly cooled properly for effectiveness to reduce failure. It would be unfortunate to fund an expensive cluster of GPUs only to learn that you can’t actually properly run it where you planned to. As a hardware and systems integrator, we don’t provide services to deploy an environment for your cluster but strive to deliver the very best service to help you avoid these mishaps by having conversations concerning these potential constraints.
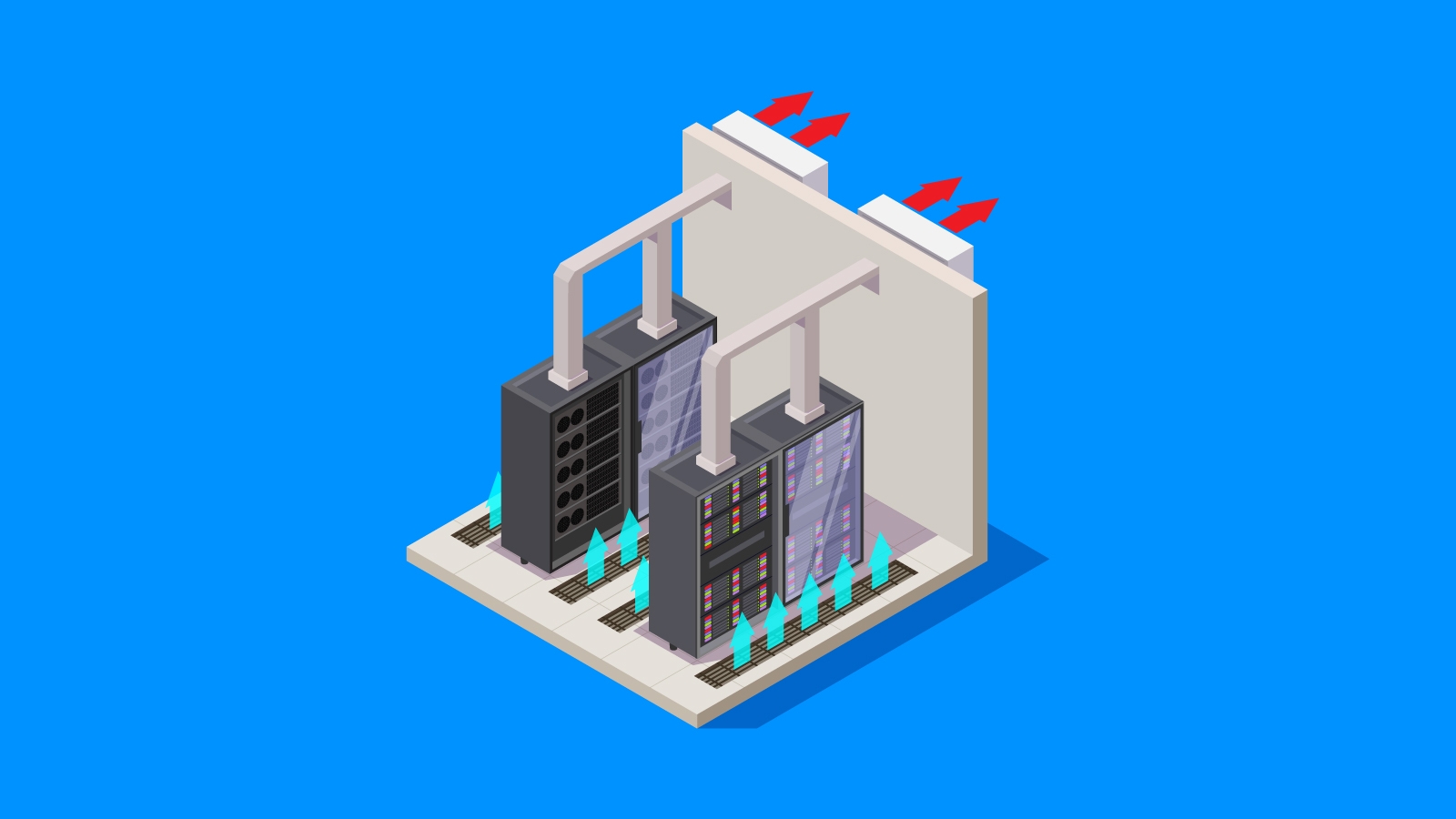
5. Budget Constraints
When looking for an AI server our customers will evaluate both on-premise and HPC cloud providers to do the job.
Cloud Service costs and services depend on providers; they are responsible for the maintenance and upkeep which is a plus but are also responsible for computing resource allocation for multiple users. This can lead to unpredictable costs, control, and security. On-premise systems provide stable, predictable costs over time, added flexibility, increase security, and a strong sense of ownership enabling you to tinker how your organization sees fit.
Budget constraints can be difficult for some vendors to work with since these systems are not close to cheap. But with a partner like Exxact, we work with you from day one to build a fully customized system and continue to deliver upgrades as your organization grows. With confidence from a vendor you can trust, it’s easier to get the right equipment faster and cheaper reliably.
Bonus Tip: Relationships
While there are many things to consider when selecting or building your very own AI GPU system it is very important to work with the right partner – one that can digest your unique business needs and provide you with a system that will perform exactly as you need it to. Whether it be with us here at Exxact or with another systems integrator, our goal is to provide resources to inspire data scientists to solve the world’s most complex problems.
With 20 years of experience providing computer hardware with an emphasis on GPUs, our engineers here at Exxact listen to the specific needs of our clients and then work with them to customize a solution, in a short time frame and within any budgetary constraints. Our AI and HPC workstations receive high praise from our clients – it’s customized, application-optimized, scalable, and delivered production-ready.
Building a workstation, an entire AI Infrastructure, or just sourcing parts?
Exxact has got you covered!
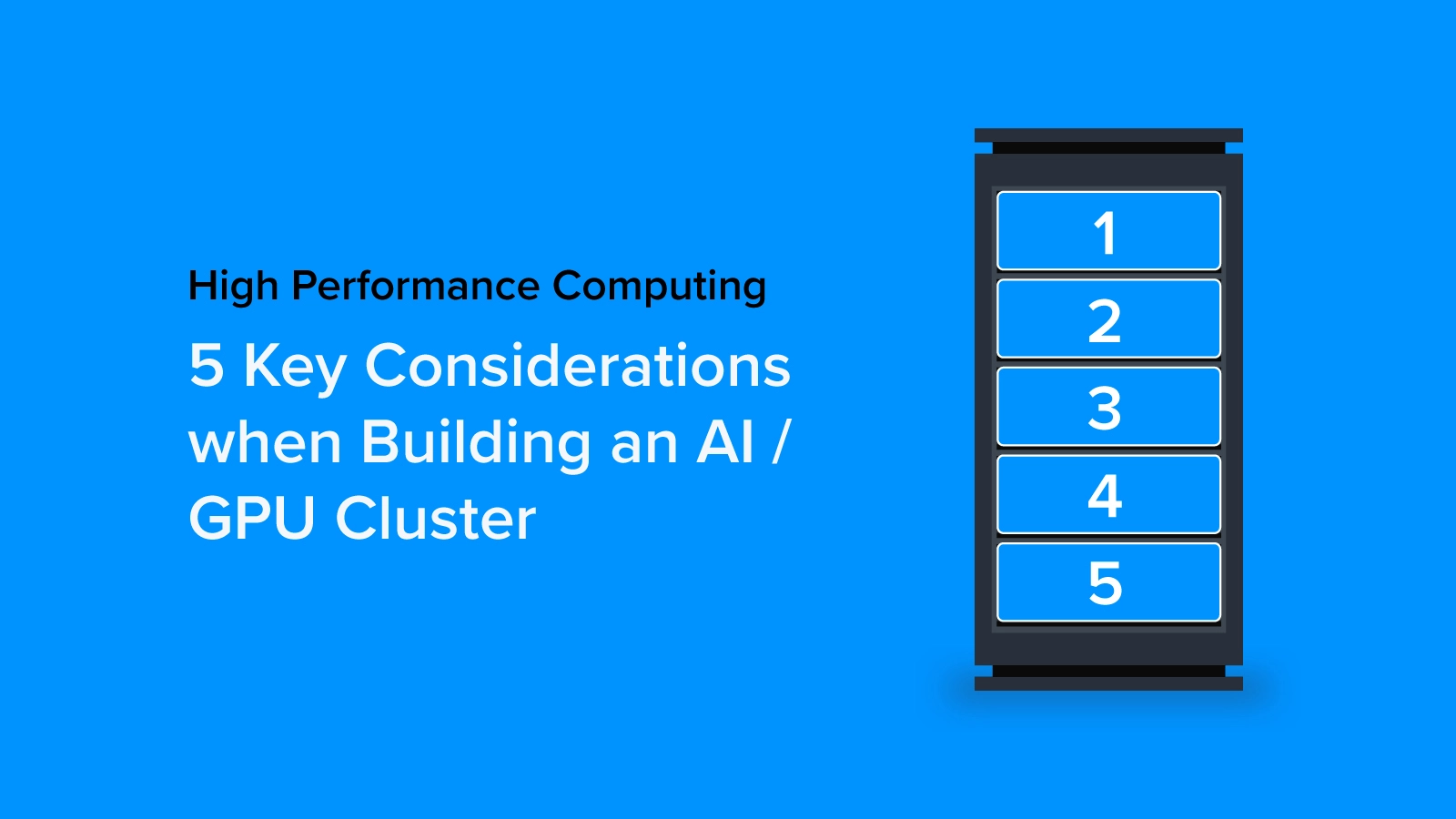
5 Key Considerations when Building an AI / GPU Cluster
As deep learning applications are constantly evolving and developing, organizations are adapting to new technologies to increase performance and capabilities. Artificial intelligence is continuing to advance the way many organizations conduct their work and research. Failing to adapt and incorporate data science technologies runs the risk of falling behind the competition.
We at Exxact want to make AI and GPU Clusters accessible and easy to implement to propel your organization to its highest potential. There is a lot going on in the world of artificial intelligence and even more to think about when building a GPU-heavy AI workstation, server, or cluster system.
GPU Clusters General Use Cases
GPUs as you may know, provide the computational power and throughput to build, train, and deploy AI models. They collect data, generate new data, analyze existing data, automate tasks, and even enhance the way we interact with the world.
Almost every industry is being impacted by the use of AI such as:
- Shopping and Advertising
- Search Engines
- Digital Personal Assistants
- Translation
- Autonomous Vehicles and Devices
- Cybersecurity
- Life Science and Healthcare
- Transportation
- Industrial Manufacturing and Automation
- Food and Farming
With such an impact on the world’s most important industries, building a GPU cluster to help develop and conceptualize an AI model to accelerate your workloads is essential to propelling your business into modernism.
5 Essentials of an AI/GPU Cluster Infrastructure
1. Applications and Industry
The types of applications you plan to run will have a significant role in how you decide to build out your system. Deep learning made large advancements being brought into the life sciences and engineering field in recent years. Be sure to consider the applications you will need to be supported in your system when you spec out the perfect artificial intelligence system. Our engineers at Exxact can help you through this critical step and the unique needs of your organization.
Machine learning (ML) has evolved quite significantly over the past decade and has ramped up over the last few years. Machine learning is the study of AI where a model learns by analyzing patterns in a given dataset with no explicit programming. Machine learning applications can help organizations solve a wide range of problems, from science to engineering. This is done through the application of trained deep neural networks and complex data science algorithms which GPUs made feasible with parallel computation.
As deep learning plays an increasingly important role in our world’s organizations, it will become more and more important day over day to consider how these technological advancements will change the field of our work and how your organization can start leveraging its power.
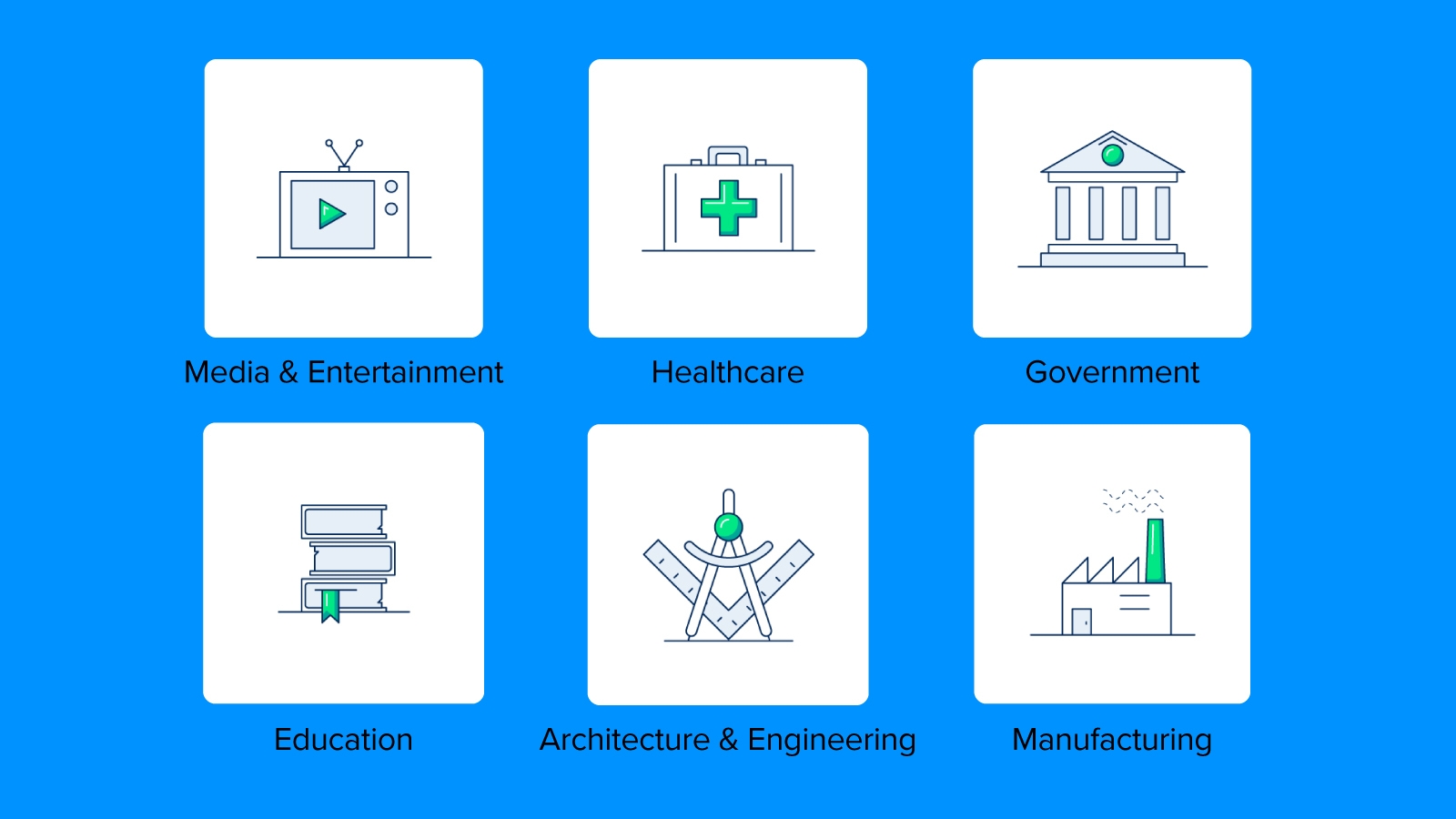
2. GPU Needs and Capabilities
When it comes to the right GPU selection, there are often so many choices to consider. Those starting out with Deep Learning and AI can get by using a competent gaming machine with a 3090 or 3090 Ti. You can also use Cloud Computing but it limits your capabilities and flexibility.
Among the most impressive GPU options is the NVIDIA A100, NVIDIA’s dedicated AI accelerator GPU built for speed and performance for scientific computing, graphics, and data analytics in data centers. NVIDIA’s next-generation NVIDIA H100 is coming to data centers in early 2023 which is claimed to have a 6x performance bump. The NVIDIA DGX Systems are the flagship and representation of peak AI computing.
The A100 and H100 are highly priced accordingly to their high efficiency, but if you’re not looking to break the bank, NVIDIA’s professional RTX lineup is a great alternative. Consider the RTX A6000, RTX A5500, or the new RTX 6000 Ada for your build.
However, if you are looking to delve straight in and need a competent machine, data center GPUs might be your best bet to save both on space, as well as increase performance per node. If you're not sure, you can always ask us!
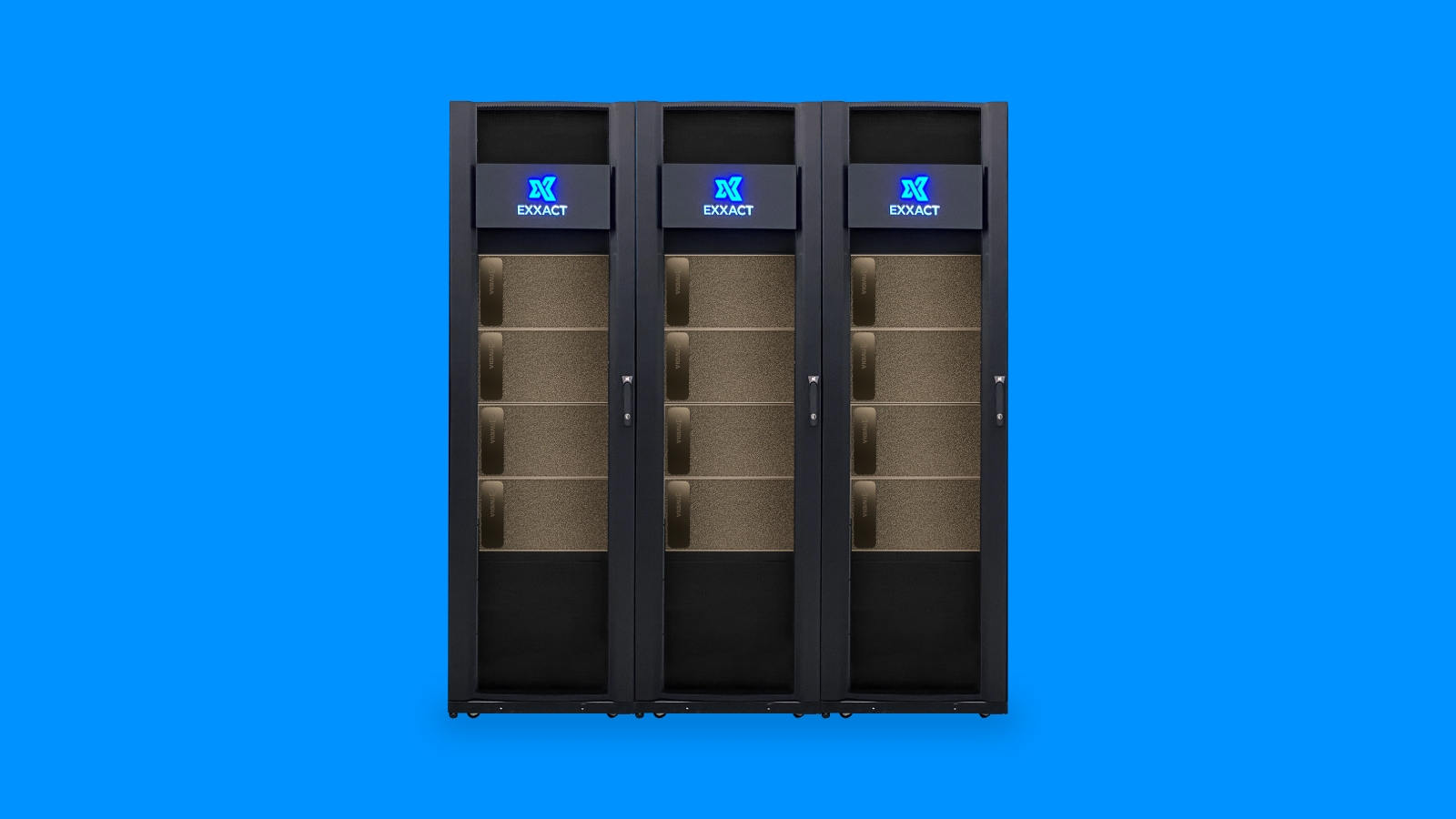
3. HPC Cluster vs. Single Server
We were hinting at it in the previous section. Consider whether you’ll need a single AI workstation a multi-GPU server or a large-scale HPC Cluster. This determination will often come down to budget constraints and the amount of data you plan to ingest, store, analyze, and process. AI/HPC server platforms offer a simple way for you to take control of your AI computing projects with maximum performance at the lowest total cost of ownership; sometimes a cluster is not always necessary when workloads are on a smaller scale.
Like the individual AI solutions (workstations and servers), our clusters come application-optimized with popular industry applications. Check out Exxact’s Supported Software page here, or ask our engineers about your specific program and we can do our best to inform you of capabilities and recommended solutions.
4. AI Infrastructure Needs
For large GPU systems, power and cooling are the main concerns. AI servers draw significantly more power than previous-generation CPU-only servers, with some of the higher-end platforms maxing out at 6000 watts. Ensuring that your facility can provide adequate power is essential in determining the size and breadth of your system.
An HVAC unit and environment are necessary to remove the heat created by these systems in operation. These GPU clusters need to be properly cooled properly for effectiveness to reduce failure. It would be unfortunate to fund an expensive cluster of GPUs only to learn that you can’t actually properly run it where you planned to. As a hardware and systems integrator, we don’t provide services to deploy an environment for your cluster but strive to deliver the very best service to help you avoid these mishaps by having conversations concerning these potential constraints.
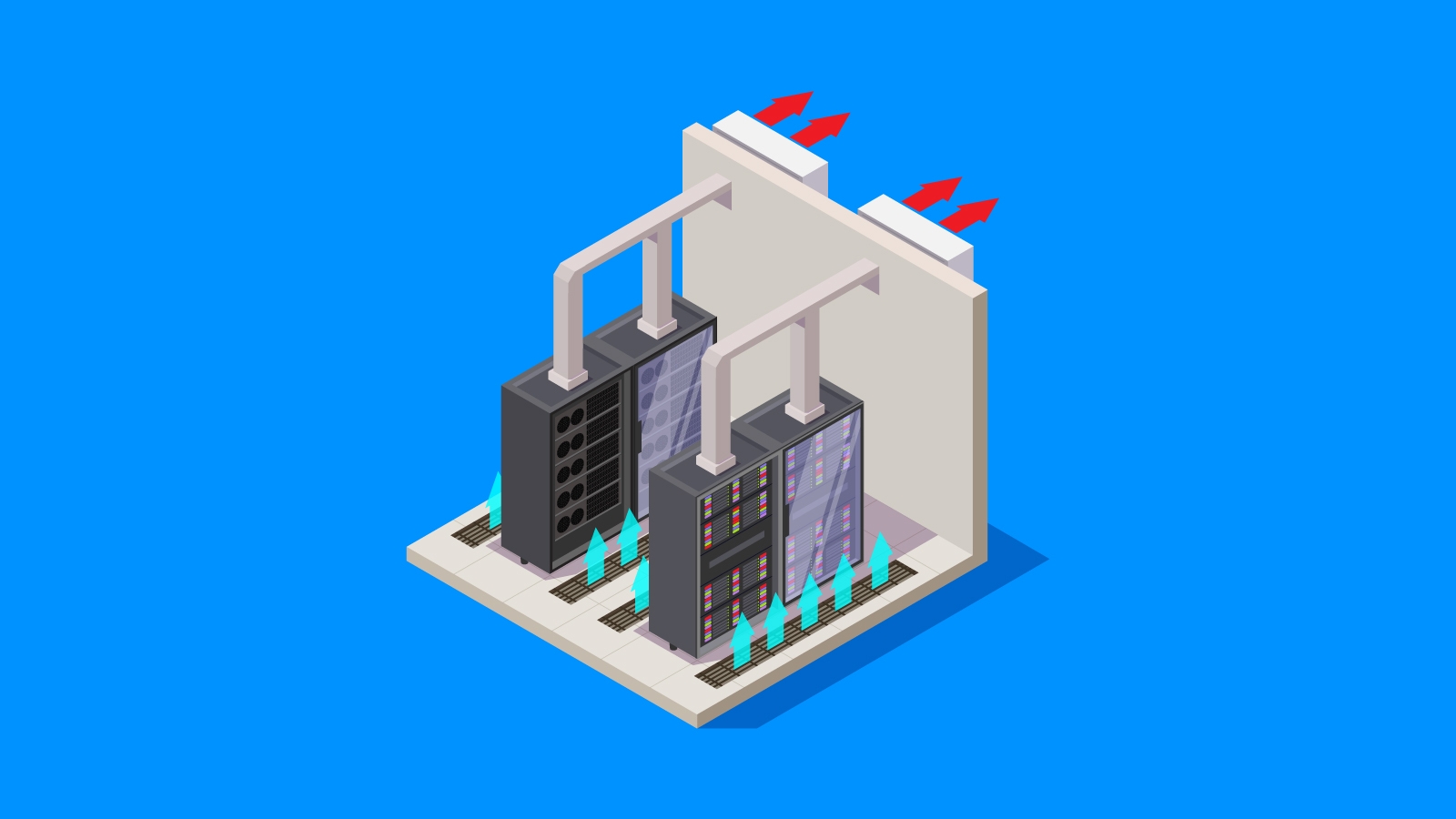
5. Budget Constraints
When looking for an AI server our customers will evaluate both on-premise and HPC cloud providers to do the job.
Cloud Service costs and services depend on providers; they are responsible for the maintenance and upkeep which is a plus but are also responsible for computing resource allocation for multiple users. This can lead to unpredictable costs, control, and security. On-premise systems provide stable, predictable costs over time, added flexibility, increase security, and a strong sense of ownership enabling you to tinker how your organization sees fit.
Budget constraints can be difficult for some vendors to work with since these systems are not close to cheap. But with a partner like Exxact, we work with you from day one to build a fully customized system and continue to deliver upgrades as your organization grows. With confidence from a vendor you can trust, it’s easier to get the right equipment faster and cheaper reliably.
Bonus Tip: Relationships
While there are many things to consider when selecting or building your very own AI GPU system it is very important to work with the right partner – one that can digest your unique business needs and provide you with a system that will perform exactly as you need it to. Whether it be with us here at Exxact or with another systems integrator, our goal is to provide resources to inspire data scientists to solve the world’s most complex problems.
With 20 years of experience providing computer hardware with an emphasis on GPUs, our engineers here at Exxact listen to the specific needs of our clients and then work with them to customize a solution, in a short time frame and within any budgetary constraints. Our AI and HPC workstations receive high praise from our clients – it’s customized, application-optimized, scalable, and delivered production-ready.
Building a workstation, an entire AI Infrastructure, or just sourcing parts?
Exxact has got you covered!