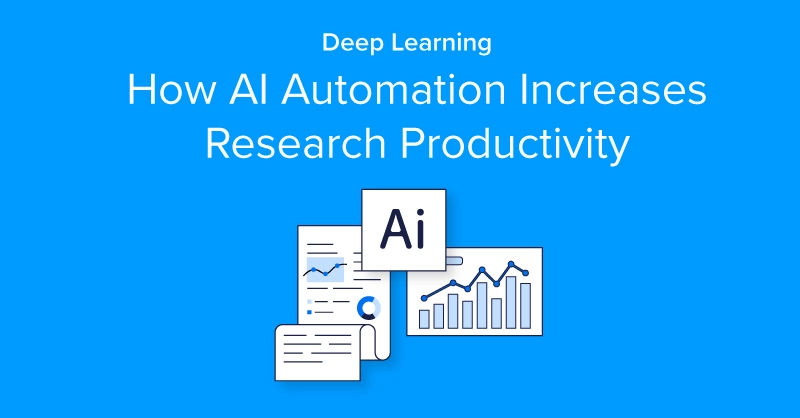
Introduction
Scientific research is about finding quick, dependable, and efficient ways to accomplish goals. As the demand for high-quality data is essential for making accurate analysis, automation has become a necessity for streamlining the workflow.
Automation is transforming research in many fields, helping with everything from collecting data to managing workflows making research faster and more productive. We explore real-life examples of automated systems that are helping businesses and researchers innovate and accelerate discoveries.
Why Automation Matters in Research Today
As research grows increasingly data-intensive automation practices provides an efficient way to process massive datasets and execute complex workflow to access insights faster. At Exxact we have seen so much need for accelerated computing solutions to power artificial intelligence (AI), machine learning (ML), and high-performance computing (HPC) workloads. GPUs and purpose-built accelerators have emerged as indispensable tools, especially for research fields that demand precision, speed, and scalability.
- Dynamic Allocation: Optimizes resource use by dynamically allocating based on workload needs.
- Real-time Monitoring: Provides instant visibility into performance, enabling proactive issue resolution.
- Cleaning Data: Executes data preprocessing upon ingest into feature rich data points to fit business workflow.
- Workflow Orchestration: Automates complex workflows like data ingestion, evaluation, and notification.
- Error Reduction: Minimizes manual errors, improving reliability and consistency.
- Accelerated Innovation: Speeds up AI/ML development, enabling faster experimentation.
Streamlining Data Collection and Preprocessing
One of the most time-intensive parts of any research project is data collection and preparation. In fields like genomics, neuroscience, and environmental science, researchers are often dealing with hundreds or even thousands of data samples requiring manual logging, cleansing, and standardizing. The data cleaning process is not only labor-intensive but also prone to errors. Automation has changed the game in multiple ways.
- Automated Data Collection: Modern automation tools enable high-speed data collection and processing, with high-throughput screening analyzing thousands of samples daily for stronger insights. IoT sensors ingest real-time data for applications like environmental monitoring and material performance, tracking variables such as temperature, humidity, and chemical composition without manual intervention. A structured approach to data ingest and preprocessing accelerates analytics, streamlining research and decision-making.
- Data Preprocessing and Quality Control: Machine learning automates data cleaning, flagging inconsistencies in real-time while GPUs preprocess vast datasets for faster insights, such as in genomics. AI-driven quality control ensures standardized, error-free data, reducing human error in screening, decision-making, and reporting. By automating these redundant tasks, AI frees up time for researchers to focus on higher-level work, making it an essential tool across industries.
- Simplifying Documentation with ELNs: Electronic Laboratory Notebooks (ELNs) automate experimental data capture, storing information in a digital, searchable format with version control and change tracking. Secure data storage prevents loss and ensures accurate reporting, while automated logging in biotech research has significantly reduced reporting errors, improving documentation and traceability.
- Workflow Management Systems: AI-driven workflow automation simplifies resource allocation, scheduling, and progress tracking, improving collaboration in large research projects. With AI’s influence expected to impact up to 80% of the U.S. workforce, by reducing manual workloads in data-intensive fields, workflow systems enable researchers to focus on innovation rather than administrative repetitive tasks.
How Automation Shaped the Modern Computing Era
The practical impact of automation in research is best illustrated by real-world applications especially in recent groundbreaking history. Here’s a closer look at some industries that have made huge impact with the help of AI.
- Pharmaceutical Research: Moderna’s COVID-19 vaccine case is a hallmark of how automation can be applied to streamline vaccine development. Automated mRNA sequence design, high-throughput screening, and robotic automation played key roles in accelerating the development process, allowing Moderna to deliver life-saving vaccines in record time.
- Environmental Research: NOAA’s uses automation in climate monitoring system to transform environmental science. By combining satellite data and automating a data cleanup to efficiently feed a machine learning, NOAA’s models can make more accurate climate predictions, crucial in addressing pressing global issues like climate change.
- Materials Science: Shell’s team leveraged NVIDIA DGX for engineering simulations such as subsurface imaging and digital rock analysis, which involves mapping pore structures in rocks. With a streamlined workflow pipeline and NVIDIA GPUs reducing high fidelity CFD simulations, Shell is able to deploy an use efficient resources to explore sustainable materials like biomass and renewable feedstocks in industrial chemistry.
Conclusion
Automation in research has fundamentally redefined productivity. By automating processes like data collection, analysis, and documentation, researchers are achieving insights faster, with greater accuracy, and at a scale that was previously unimaginable. But it’s not just about speed—automation enables researchers to explore deeper, innovate further, and address global challenges with precision.
- Increased Integration of AI and ML: AI-driven experimental design and hypothesis generation will make research smarter and more proactive, driving new discoveries by modeling complex systems.
- Advanced Computing Resources: Accelerated computing could unlock new possibilities for complex simulations, especially in drug discovery and materials science.
- Enhanced Collaboration Tools: The future promises more interconnected research platforms, enabling real-time data sharing and cross-institutional collaboration on a global scale.
The path forward lies in striking a balance between the benefits of automation and the irreplaceable value of human insight. Human creativity and automated efficiency can propel research into a new era of discovery and impact, one where innovation isn’t limited by time or scale but empowered by innovators and AI automations working harmoniously. AI agents have become a huge topic in the AI era for developing agentic AI that can plan and execute decisions based on a prompted goal. If you have questions on how your business can leverage the power of AI through accelerated computing, Exxact offers configurable computing infrastructures that fits any deployment size from GPU workstations to full scale data centers. Contact us to learn more.
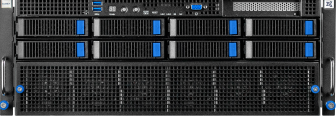
Fueling Innovation with an Exxact Multi-GPU Server
Training AI models on massive datasets can be accelerated exponentially with the right system. It's not just a high-performance computer, but a tool to propel and accelerate your research.
Configure Now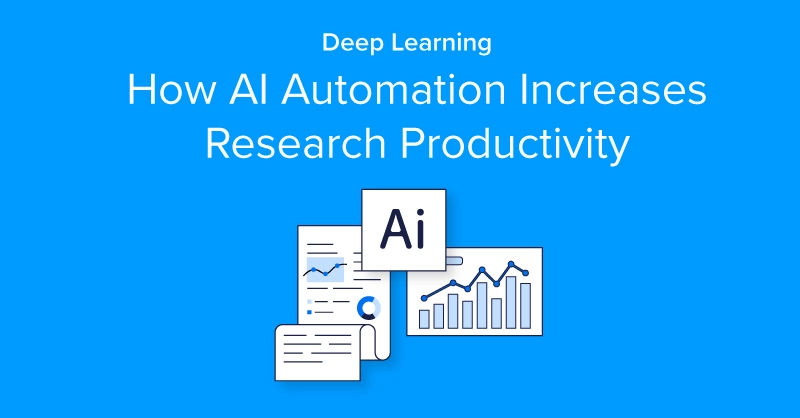
How AI Automation Increases Research Productivity
Introduction
Scientific research is about finding quick, dependable, and efficient ways to accomplish goals. As the demand for high-quality data is essential for making accurate analysis, automation has become a necessity for streamlining the workflow.
Automation is transforming research in many fields, helping with everything from collecting data to managing workflows making research faster and more productive. We explore real-life examples of automated systems that are helping businesses and researchers innovate and accelerate discoveries.
Why Automation Matters in Research Today
As research grows increasingly data-intensive automation practices provides an efficient way to process massive datasets and execute complex workflow to access insights faster. At Exxact we have seen so much need for accelerated computing solutions to power artificial intelligence (AI), machine learning (ML), and high-performance computing (HPC) workloads. GPUs and purpose-built accelerators have emerged as indispensable tools, especially for research fields that demand precision, speed, and scalability.
- Dynamic Allocation: Optimizes resource use by dynamically allocating based on workload needs.
- Real-time Monitoring: Provides instant visibility into performance, enabling proactive issue resolution.
- Cleaning Data: Executes data preprocessing upon ingest into feature rich data points to fit business workflow.
- Workflow Orchestration: Automates complex workflows like data ingestion, evaluation, and notification.
- Error Reduction: Minimizes manual errors, improving reliability and consistency.
- Accelerated Innovation: Speeds up AI/ML development, enabling faster experimentation.
Streamlining Data Collection and Preprocessing
One of the most time-intensive parts of any research project is data collection and preparation. In fields like genomics, neuroscience, and environmental science, researchers are often dealing with hundreds or even thousands of data samples requiring manual logging, cleansing, and standardizing. The data cleaning process is not only labor-intensive but also prone to errors. Automation has changed the game in multiple ways.
- Automated Data Collection: Modern automation tools enable high-speed data collection and processing, with high-throughput screening analyzing thousands of samples daily for stronger insights. IoT sensors ingest real-time data for applications like environmental monitoring and material performance, tracking variables such as temperature, humidity, and chemical composition without manual intervention. A structured approach to data ingest and preprocessing accelerates analytics, streamlining research and decision-making.
- Data Preprocessing and Quality Control: Machine learning automates data cleaning, flagging inconsistencies in real-time while GPUs preprocess vast datasets for faster insights, such as in genomics. AI-driven quality control ensures standardized, error-free data, reducing human error in screening, decision-making, and reporting. By automating these redundant tasks, AI frees up time for researchers to focus on higher-level work, making it an essential tool across industries.
- Simplifying Documentation with ELNs: Electronic Laboratory Notebooks (ELNs) automate experimental data capture, storing information in a digital, searchable format with version control and change tracking. Secure data storage prevents loss and ensures accurate reporting, while automated logging in biotech research has significantly reduced reporting errors, improving documentation and traceability.
- Workflow Management Systems: AI-driven workflow automation simplifies resource allocation, scheduling, and progress tracking, improving collaboration in large research projects. With AI’s influence expected to impact up to 80% of the U.S. workforce, by reducing manual workloads in data-intensive fields, workflow systems enable researchers to focus on innovation rather than administrative repetitive tasks.
How Automation Shaped the Modern Computing Era
The practical impact of automation in research is best illustrated by real-world applications especially in recent groundbreaking history. Here’s a closer look at some industries that have made huge impact with the help of AI.
- Pharmaceutical Research: Moderna’s COVID-19 vaccine case is a hallmark of how automation can be applied to streamline vaccine development. Automated mRNA sequence design, high-throughput screening, and robotic automation played key roles in accelerating the development process, allowing Moderna to deliver life-saving vaccines in record time.
- Environmental Research: NOAA’s uses automation in climate monitoring system to transform environmental science. By combining satellite data and automating a data cleanup to efficiently feed a machine learning, NOAA’s models can make more accurate climate predictions, crucial in addressing pressing global issues like climate change.
- Materials Science: Shell’s team leveraged NVIDIA DGX for engineering simulations such as subsurface imaging and digital rock analysis, which involves mapping pore structures in rocks. With a streamlined workflow pipeline and NVIDIA GPUs reducing high fidelity CFD simulations, Shell is able to deploy an use efficient resources to explore sustainable materials like biomass and renewable feedstocks in industrial chemistry.
Conclusion
Automation in research has fundamentally redefined productivity. By automating processes like data collection, analysis, and documentation, researchers are achieving insights faster, with greater accuracy, and at a scale that was previously unimaginable. But it’s not just about speed—automation enables researchers to explore deeper, innovate further, and address global challenges with precision.
- Increased Integration of AI and ML: AI-driven experimental design and hypothesis generation will make research smarter and more proactive, driving new discoveries by modeling complex systems.
- Advanced Computing Resources: Accelerated computing could unlock new possibilities for complex simulations, especially in drug discovery and materials science.
- Enhanced Collaboration Tools: The future promises more interconnected research platforms, enabling real-time data sharing and cross-institutional collaboration on a global scale.
The path forward lies in striking a balance between the benefits of automation and the irreplaceable value of human insight. Human creativity and automated efficiency can propel research into a new era of discovery and impact, one where innovation isn’t limited by time or scale but empowered by innovators and AI automations working harmoniously. AI agents have become a huge topic in the AI era for developing agentic AI that can plan and execute decisions based on a prompted goal. If you have questions on how your business can leverage the power of AI through accelerated computing, Exxact offers configurable computing infrastructures that fits any deployment size from GPU workstations to full scale data centers. Contact us to learn more.
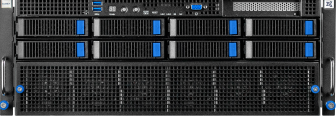
Fueling Innovation with an Exxact Multi-GPU Server
Training AI models on massive datasets can be accelerated exponentially with the right system. It's not just a high-performance computer, but a tool to propel and accelerate your research.
Configure Now